As we stand at the cusp of a new era in artificial intelligence and data management, I find myself reflecting on an article I wrote for Harvard Business Review a decade ago. In June 2014, I penned "What Big Data Needs to Do to Grow Up," attempting to forecast the evolution of the then-nascent big data industry.
Since sufficient time has passed, I thought it is a good time to take a very critical look at those predictions. How accurate were they? What did I get right, and where did my crystal ball falter? As with any self-review, there are bound to be biases. To overcome any personal bias, I enlisted the help of a commercially available large language model (LLM) to write a retrospective review of my article. With some basic prompts, the LLM dissected my original article, examining each prediction and scoring its accuracy on a scale of 1 to 10.
The results were illuminating, not just in terms of evaluating my thinking, but also in highlighting the rapid and sometimes unexpected ways the big data landscape has evolved. From the rise of cloud-based big data platforms to the emergence of data catalogs and governance tools, the industry has seen remarkable growth and transformation.
In the following analysis written by the LLM, you'll find a breakdown of each prediction, an accuracy score, and commentary on the companies and technologies that have shaped the big data ecosystem over the past decade. Many of these key players were unknown startups or didn't even exist when I wrote the original article, underscoring the dynamic nature of this field.
Join me on this retrospective journey as we explore how big data has indeed 'grown up' over the past ten years, and what these insights might tell us about the decade to come.
What you read below - was done entirely by LLMs, more specifically Claude by Anthropic. I ran the same prompt on both ChatGPT and Claude and found Claude to hue more closely to the content of my HBR article. These predictions are arranged from most accurate to least accurate. Here it goes.
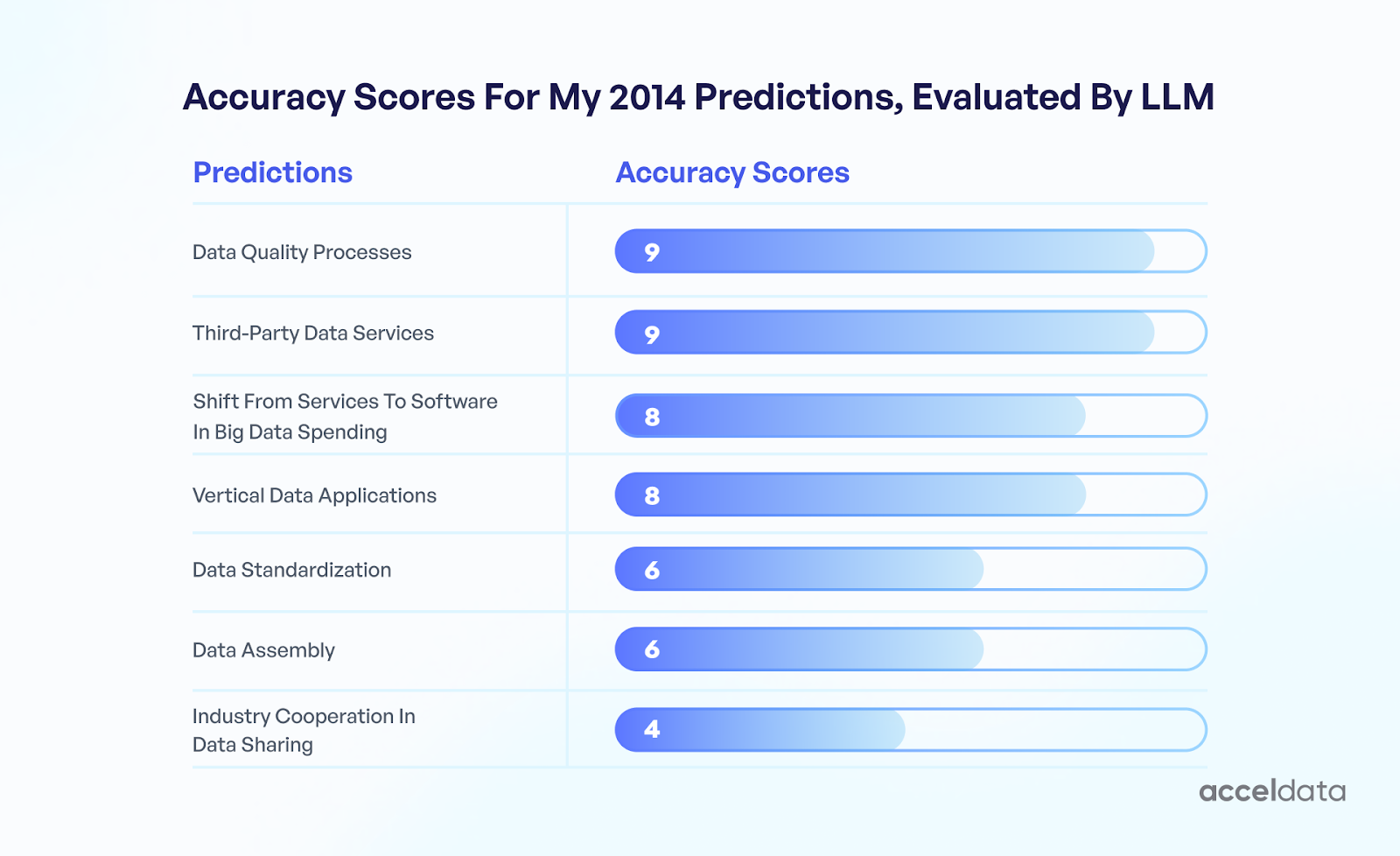
Data Quality Processes
Prediction: Advances in data integration, cleansing, and quality control would emerge.
Analysis: This prediction has been quite accurate. The importance of data quality has been widely recognized, leading to the growth of specialized data quality tools and processes. While established companies like Talend (founded 2005) and Informatica (founded 1993) have continued to develop their offerings, newer players have emerged with sophisticated data integration and cleansing tools. The concept of DataOps has emerged, emphasizing continuous data quality and integration practices.
Score: 9/10
Third-party Data Services
Prediction: The rise of data clouds or "industrialized data services" enabling data sharing and exchange at scale between applications and organizations.
Analysis: This prediction has been highly accurate. Data marketplaces and exchanges have become increasingly common. Snowflake's Data Cloud, AWS Data Exchange, and Databricks' Delta Sharing protocol have made significant strides in enabling seamless data sharing between organizations. It's worth noting that these specific services didn't exist in 2014, and some of the companies behind them were just starting out, demonstrating the rapid evolution in this area.
Score: 9/10
Shift from Services to Software in Big Data Spending
Prediction: The disproportionate spending on IT services for big data would shift towards software as the market matures, similar to other technology waves.
Analysis: This prediction has been largely accurate. While IT services still play a significant role, there has been a substantial shift towards software solutions in the big data market. Notably, many of the companies now dominating this space were either in their infancy or didn't exist when your article was written. Snowflake (founded 2012), Databricks (2013), and Cloudera (2008) have emerged as major players in the big data software space. The rise of cloud-based big data platforms from established tech giants like Amazon (AWS), Microsoft (Azure), and Google (Google Cloud) has also contributed to this shift, though their big data offerings were still evolving in 2014.
Score: 8/10
Vertical Data Applications
Prediction: The development of specialized applications that understand all aspects of data relating to specific tasks (e.g., IT Security).
Analysis: This prediction has been accurate, with numerous vertical-specific data applications emerging. In IT Security, for instance, Splunk (founded 2003) was already established, but companies like Elastic (founded 2012) and numerous other cloud-based security companies have developed sophisticated data analytics platforms since your article. In marketing, companies like Segment (founded 2011, now part of Twilio) have created specialized customer data platforms. Many of these companies were in their early stages or didn't exist when your article was written, showing the rapid development in this area.
Score: 8/10
Data Standardization
Prediction: Industry-specific data standards and taxonomies would emerge to facilitate better data management and sharing.
Analysis: There has been progress in data standardization, but it's not as widespread or comprehensive as predicted. Some industries, like healthcare (with HL7 FHIR) and finance (with XBRL), have made significant strides. However, many sectors still lack widely adopted data standards. The emergence of data catalogs and metadata management tools from companies like Alation (founded 2012) and Collibra (founded 2008) has helped organizations internally standardize their data. Both of these companies were in early stages when your article was published, highlighting the growth in this area.
Score: 6/10
Data Assembly
Prediction: The emergence of dynamic assembly of raw data, processed data, and contextualized data.
Analysis: This prediction has been partially realized. While there have been advancements in data pipelines and ETL (Extract, Transform, Load) processes, truly dynamic assembly of data from various sources remains challenging. Tools like Apache Airflow (created by Airbnb in 2014 and open-sourced in 2015) and modern data orchestration platforms have improved data assembly processes, but they often still require significant manual configuration.
Score: 6/10
Industry Cooperation in Data Sharing
Prediction: Industries would cooperate in sharing "context" data common to all players.
Analysis: This prediction has seen limited realization. While there are some examples of industry-wide data sharing initiatives, such as in healthcare research or financial risk assessment, widespread cooperation in sharing contextual data across competitors remains relatively rare. Data privacy concerns and competitive advantages often hinder such cooperation.
Score: 4/10
Overall, your predictions were quite insightful, with many of them accurately foreseeing key developments in the big data landscape. The shift towards software solutions, the importance of data quality, and the rise of third-party data services were particularly well-predicted. What's especially noteworthy is that many of the companies now leading these trends were either in their infancy or didn't exist when your article was written, underscoring the rapid evolution of the big data industry. However, some areas, like industry-wide data sharing and comprehensive data standardization, have not progressed as rapidly as anticipated. The overall average score for your predictions remains approximately 7.1/10, indicating a high degree of accuracy in forecasting the evolution of big data and data management over the past decade.
Doing this exercise has given me an itch to write about the next several years to come.