This blog is adapted from a session at the recent Enterprise Data Summit, “Data management and analytics market trends: Rosy or cautiously optimistic?,” hosted by Acceldata CEO and Co-founder Rohit Choudhary, and George Mathew, Managing Director at Insight Partners. We invite you to watch the session as well.
The session covers a range of topics about the current state and future trends related to AI, LLMs, and the role that data observability plays in fueling innovative ways to productize and innovate with these technologies. These gentlemen use their knowledge and experience in the data field to identify themes that will play out as the field of generative AI evolves. Among the topics detailed in the discussion are:
- The emergence of industry-specific LLMs and the role they play in creating more accurate models
- The balance that will need to be created between use of public and private data to strengthen LLMs
- Investment trends in AI and where the market is heading
- The challenges of operationalizing AI
- Importance of monitoring for accuracy in model-based outcomes
- Why data reliability and data observability will dictate the success or failure of LLMs
Generative AI is Having a Moment
Rohit Choudhary: When we look at AI, particularly, generative AI, we have to question: is this a web moment, an Internet moment, or a protocol moment, or is this the advent of the cloud as a service moment? How would you characterize this?
George Mathew: Yeah, I think it's good to frame it in the context of some of these larger disruptive innovations that have happened in the market for a good part of 25, 30 plus years in computing.
Before that, we saw a number of technical innovations, particularly around the advent of mobile computing, and then the introduction of the iPhone. And then subsequently after that, the cloud began to scale in the mid-2010s. I look at this as a very similar moment and probably more on the scale of where the web was back in the mid-1990s.
The reason I mentioned that as the closest analogy is that the web evolved as an artifact of how you communicate and connect all the parts of your personal and professional life in a way that we hadn't been able to before the mid-90s. It really transformed enterprises and society.
And I think that's the level of scale and opportunity that we're seeing here in the use of generative AI, particularly when it comes to both enterprises and the use of narrow AI techniques, particularly in terms of improving the software experiences. But there’s also the potential for profound change in how humanity can achieve some level of artificial general intelligence that would have a significant impact on society itself. And all that's going to play out in the next decade or two. And so in my frame of reference, it feels as big and as important as the World Wide Web was back in the mid-90s.
Rohit Choudhary: It's a big shift from where we were, from being very procedural to being guided by what is necessary. And it’s quite a shock to consider what will be produced by generative AI, because the quality can be surprisingly good, and it ultimately opens up tremendous possibilities.
More code can be written, more use cases can be solved, more data can be generated. Do you think that the world is prepared to understand the consequences of what this might mean? And particularly if you could contextualize that from a data management perspective. What would it mean for enterprise leaders who are shaping up right now and thinking through their AI strategy.
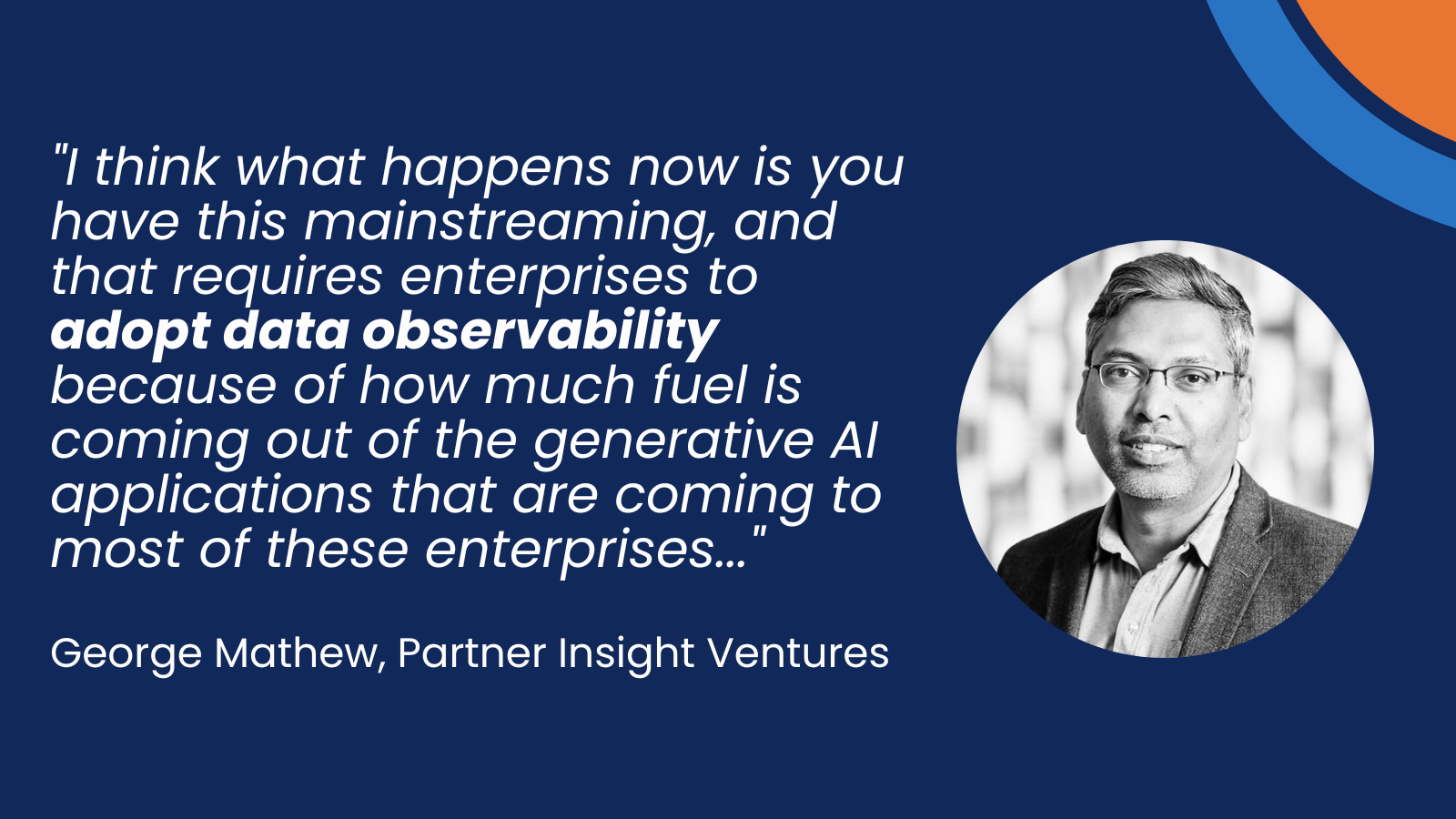
George Mathew: Think about it this way - why is this different from just a pure use of the analytics capabilities of AI and ML?
Much of what has been developed has been on the predictive side by using techniques like machine learning and particularly deep learning techniques that are the foundation of where these large language models (LLM) have done incredibly well in the broader context of a transformer-based architecture.
Neural-based learning as a subdomain provides an opportunity to bring more data into the models. They’re providing an almost human-like response in terms of model performance and the model's ability to do human-like work.
And that's the profound shift because we’re moving from predicting things to creating things. We’re no longer saying, hey, this is what we predict is going to happen to this particular piece of data or this particular insight that we want to generate around the business. Instead, we can say, write me a blog post or create a new image that places my product in Brazil using just the prompt interface and your basic image. The rest of it is generatively constructed and that is affecting all kinds of work in a way that has been unimagined even as little as 18 months ago.
Think about what that means for the way enterprises work today. Most workflows and experiences that we've confined from a non-generative standpoint up to this present moment have been radically reimagined, or they have the potential of being radically reimagined in the next half a decade, perhaps even sooner. Underpinning all that is the fact that data is the substrate that's really making these generative models really work.
At scale, a lot of that data is being provided through public corpus-like information that is scraped from remarkable online sources. At scale, 20% of human knowledge that's on the web today has gone into creating GPT 4; it’s likely somewhere in the neighborhood of a trillion parameter large language model. Over time, we’re going to recognize that private data is going to be quite important.
What I've been thinking about from an investment standpoint is how much more these private data opportunities will drive the next decade of capability around generative AI, beyond sucking in a large swath of publicly available information, which is the underpinning of language and reasoning and understanding. But the domain specificity is all going to come from the private data.

What Comes Next? The Importance of Private Data
Rohit Choudhary: That's right. I think the access to this private proprietary data is going to just add so much more to the accuracy of what is being produced and in the context of what has to be done. For example, in healthcare, finance, and legal, I think we're already seeing some massive shifts. And you're absolutely right that earlier we went from doing some categorization taxonomy prediction to now actually generating things that are really exciting.
George Mathew: As you mention, these industry-domain models are rapidly emerging. Bloomberg started developing their LLM, Bloomberg GPT only about two or three months ago. It’s focused on the domain of financial reporting. Harvey AI is building one for the legal market. Hippocratica, one of our recent investments, was made to understand medical literature better and leveraging a generative model for that
Rohit Choudhary: There are many ways to understand the future, and one lens is through where AI startups are putting their energy. Can you talk with us about what you’re seeing in terms of new opportunities, where you see the areas that are most primed for disruption?
My sense is that it could start with consumer uses, move into utilities, and then eventually head into the enterprise. Is that the right way to think about it?
George Mathew: Yes, I think that's right. In the current realm of investing, particularly around foundation models and generative AI, let's be clear that this is all built on a data substrate. So the modern data stack has been an essential part of how we see enterprise-based generative model work occurring and just foundation models being built. So particularly as the need for private data is going to become more and more important over time.
For generative AI in particular, we're noticing that a lot of the investments and the innovations are happening in terms of full stacks that are being purpose-built for a specific persona. Additionally, we're seeing tools emerge for LLM and foundation model operations, as well as LLM orchestration tools that are being used to build ensemble-based models.
For enterprise data orchestration and for enterprise data observability, you're going to see a fair amount of fulfilling the needs of building this modern data substrate. And we certainly are seeing the importance from data observability, data pipeline, and data quality perspectives, which Acceldata has led efforts in over these last several years.
Think about how many more tailwinds are emerging because you're building these generative applications and you have to really understand the data quality and the orchestration that surrounds just getting just high quality observability, particularly from a data pipeline standpoint.
The thinking goes like this: if you have bad quality data going into a generative model, it hallucinates. The hallucinations are only reduced by ensuring that there's high quality data that is appropriately being monitored and observed as it's going through a variety of data pipelines. And that's not getting any more straightforward. In fact, it's actually getting more significantly complex as an opportunity for anyone who's in the data observability market.
The Critical Importance of Data Reliability and Data Observability for AI
Rohit Choudhary: When we talk to data leaders, it appears that they’re sitting at an intersection of three problems:
- Talent: this problem is never going to solve itself. Do you have enough talented people across the data analytics and now machine learning and AI pipeline?
- Technology selection: teams need to determine if they’ll go with private or public models.
- Data delivery: for data supply chains, what is the operability model? How do we build them for today, but also for 18 months from now, and five years from now?
George Mathew: For the talent issue, consider that the primary benefit of some of the foundation models and the LLMs are that they introduce much more significantly capable natural language interfaces today to how software runs and works.
So in that sense, there is just this general democratization and lowering the bar of how many more people have access to complex capable software that you don't have to have a large instruction manual to be able to run the software itself. You have these more democratized experiences coming to a table near us, mainly because of the generative AI capabilities that are driving that democratization.
But while that's happening, you still need to be able to deliver high quality data to those endpoints, whether those endpoints be your cloud native data lakes and data warehouses, whether that be some of the applications that are naturally built on top of that operational substrate. And in most of those cases, that complexity in terms of what your data pipelines are, are not going to somehow be reduced over time.
And a lot of ways that means that you just need better observability. In a lot of ways you and the team at Acceldata really defined that capability, and I think what happens now is you have this mainstreaming, and that requires enterprises to adopt the need around data observability because of how much fuel is coming out of the generative AI applications that are coming to most of these enterprises.
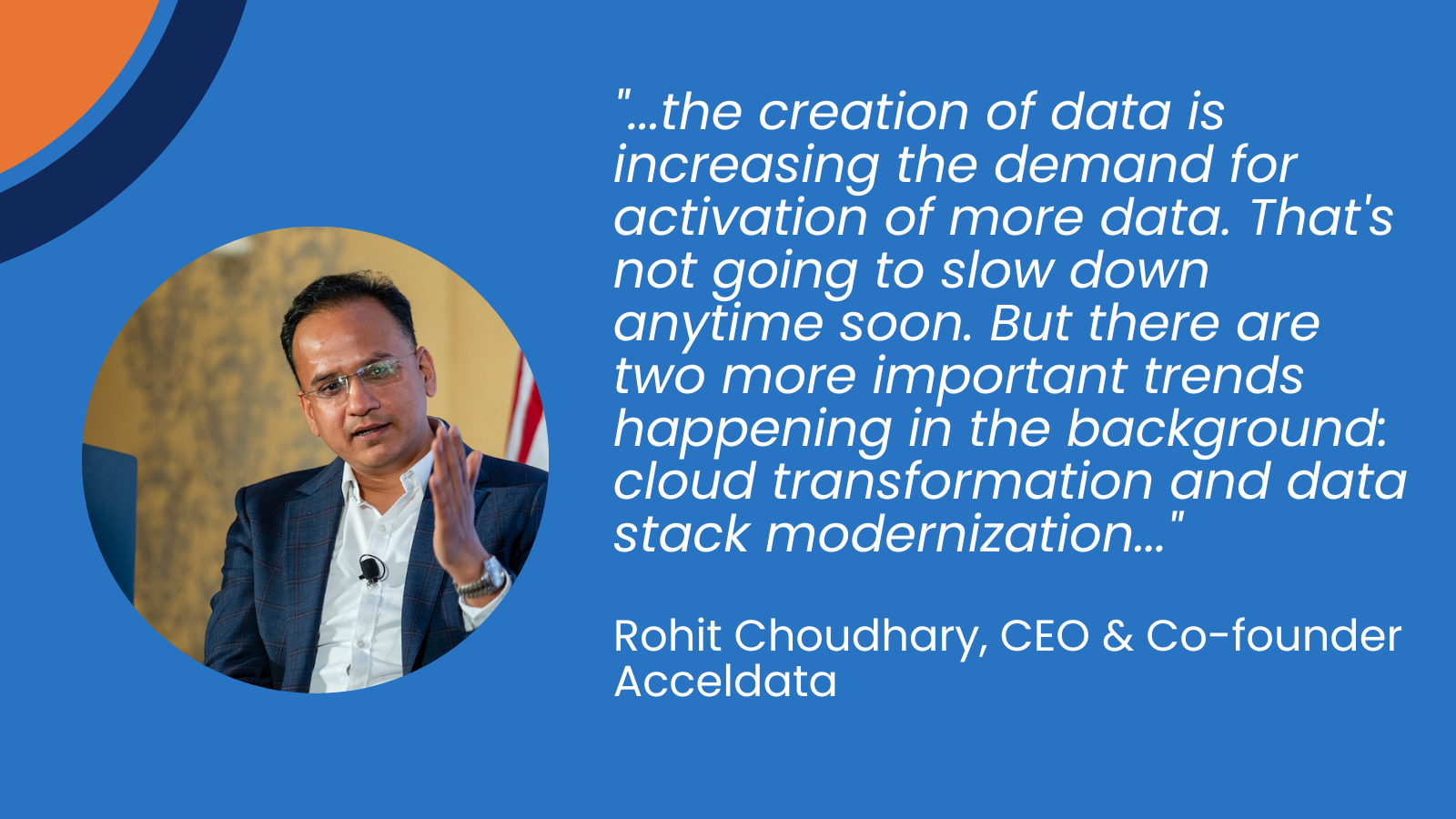
Rohit Choudhary: The creation of data is increasing the demand for activation of more data. That's not going to slow down anytime soon. But there are two more important trends happening in the background: cloud transformation and data stack modernization.
All of that requires an immense amount of monitoring because these are hundreds of millions of dollars being put into these two large efforts annually. And people would like to know what the results are. And when you're trying to get to high quality outcomes, everything needs to be monitored. I just keep telling people that if you have a few gold bars and you had it in a locker, would you not monitor that all the time?
And when you have data, then you're trying to continuously look at whether the outcomes are right or not, especially when it has the potential to change outcomes. In other words, the report being wrong is not just a report being wrong. It essentially means that the pipeline is broken and nobody has paid any attention to it. But if you’re working in a bank and today is when reconciliation happens, you're all messed up.
George Mathew: Imagine how much the stakes have increased now, right? It used to be like, yeah, maybe that report is wrong, but I can figure out why it's wrong and I have enough context around me. There might be a business user, a non-technical person in the midst of this that's literally interfacing with a chat-like UI, and asking a question and a generative response comes back and it looks authoritative and right.
But in reality, if you didn't get enough high quality data into that application, you're going to see some level of unintended corporate hallucination in your chat bot being powered by a generative model. If you don’t have high quality data, all kinds of messy mistakes can happen.
The Impact of AI on Data Experiences
Rohit Choudhary: Let’s close this with one last question. How are boards reacting to gross margins being affected by the use of LLMs and the amount of compute that companies will need today and in the future?
And more fundamentally, is compute available today for enterprises? And the last bit of that question, the tail of that question is that is this the final need for all the enterprises to then start moving to the cloud? Is this going to be that huge massive push towards the cloud?
George Mathew: The level of attention by boards for LLMs and their impact has definitely reached peak buzz. We're seeing value being created both in new product strategies as well as efficiencies in how the business can achieve certain levels of just better optimization.
We're now seeing several thousand person organizations now being shrunk to 200 people and a generative model. So there are great opportunities for growth and efficiency that are happening simultaneously with the use of generative models, where they are reimagining how enterprises run and how software businesses are built. Over the long run, I think this does accelerate the movement to the cloud.
In the short term, it will introduce more high-priced, premium products built on top of generative AI tools. Some of those are already getting as much as 30-40% premiums on them. This isn’t just what Chat GPT has done recently with their premium offering, but also the kinds of things coming from Microsoft and Salesforce, and others.
We’re going to see a radical movement towards really driving AI as a fundamental part of software experiences, and how enterprises run. But that's where I just think that this is just going to be just the way that everyone works. And that's a pretty exciting future for anyone in the enterprise today, and certainly anyone who's really driving the next generation of observability, particularly an enterprise like you and the team have been at Acceldata.
Watch Rohit and George's Session on AI and Data Management Trends
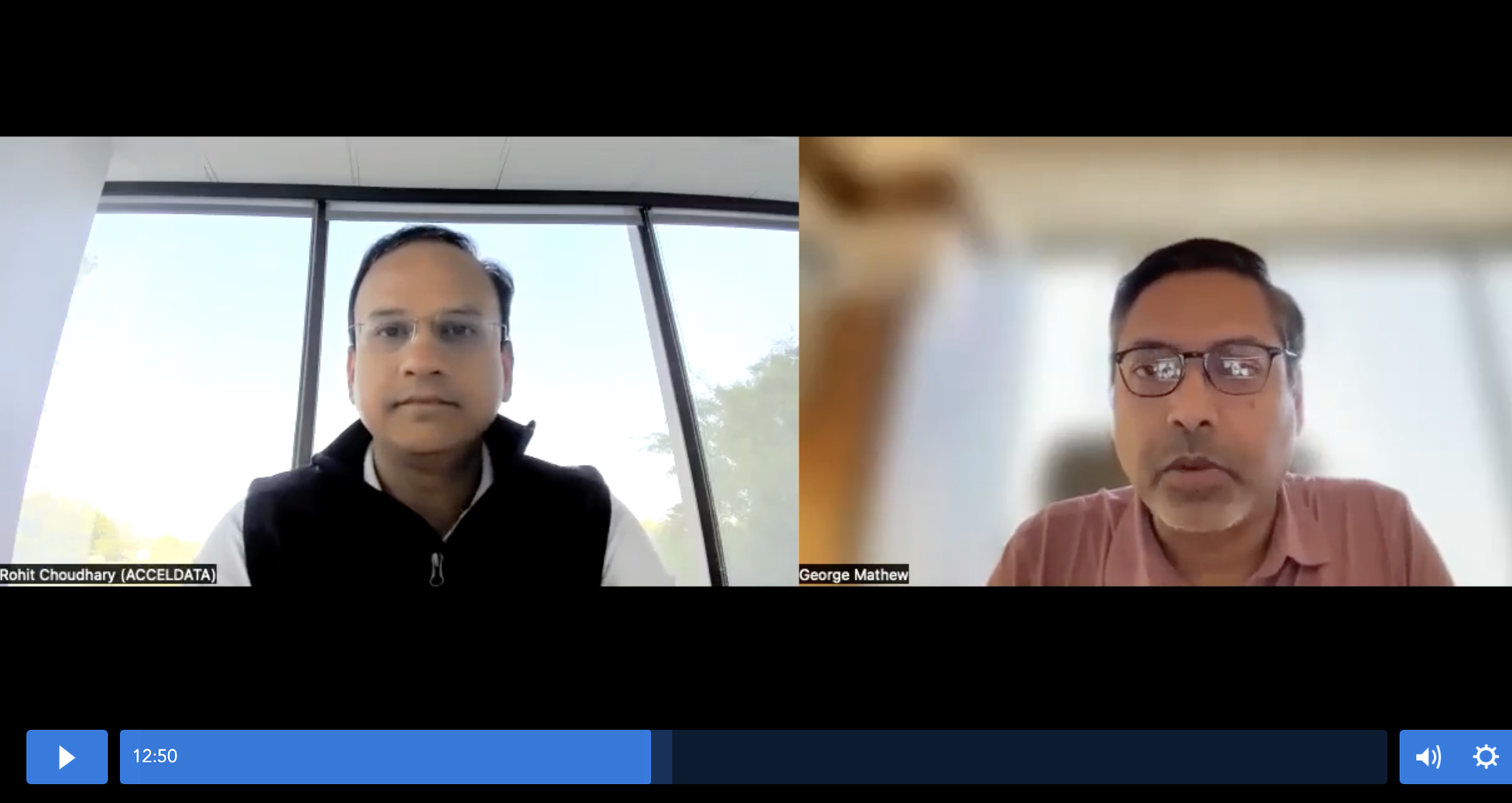