Data Observability is gaining significant interest and adoption in organizations that manage large, complex data environments. It is an extension of the Observability trend that has modernized Application Performance Monitoring (APM).
Observability is a measure of how well internal states of a system can be inferred from knowledge of its external outputs. The idea is that new, actionable insights can be gained to improve a complex system with a combination of monitoring (external outputs) and analytics (inference).
Data Observability
An observability strategy can be applied to complex data operations to drive improvements in data, processing and pipelines. The approach emphasizes three key elements:
- Monitor: Data Observability begins with capturing many different external outputs from data operations such as performance metrics, metadata, utilization, etc. This enables a 360-degree view to identify both known and unknown concerns and opportunities.
- Analyze: Data observability then analyzes the data and correlates these observations into meaningful intelligence that allows users to interpret what is happening inside opaque systems. This provides a wealth of insight that can be used to make data more reliable, scalable and cost effective.
- Act: When insights are uncovered you can then inform or take automated action through a data observability platform and/or other engineering approaches and technologies to rectify immediate issues, avoid future ones and optimize for efficiency.
There are three primary areas where Data Observability complements or exceeds existing technology: Compute Performance Monitoring, Data Quality and Governance, and Data Pipeline Management.
Performance Monitoring
Tools exist to monitor many technology layers: infrastructure, networking, apps, security, and so on. Data Observability provides advanced performance monitoring capabilities to ensure that data processing is reliable, performant, and efficient. Examples of performance monitoring that are limited or non-existent elsewhere include:

Data Operations and Management
Data governance technologies such as data catalogs and data quality tools often require granular, manual work and focus on addressing known or expected issues. Many Data Observability capabilities overlap with existing data governance technologies but typically go beyond in several key areas.
A Data Observability approach to data governance can monitor for a broader range of data risks, reduce manual effort through automation, and achieve greater precision through machine learning.
Anomalies are often detected when it comes to data movement, quality, structural change (schema drift), and data trends (data drift). When these anomalies are observed, the traditional tools and approaches (which can be more labor intensive) can be applied to address the issue.
In summary, both the amount of labor and unknown risks make the case for an approach where you Observe everything and Engineer only as needed.
Examples of Data Observability supporting data governance include:
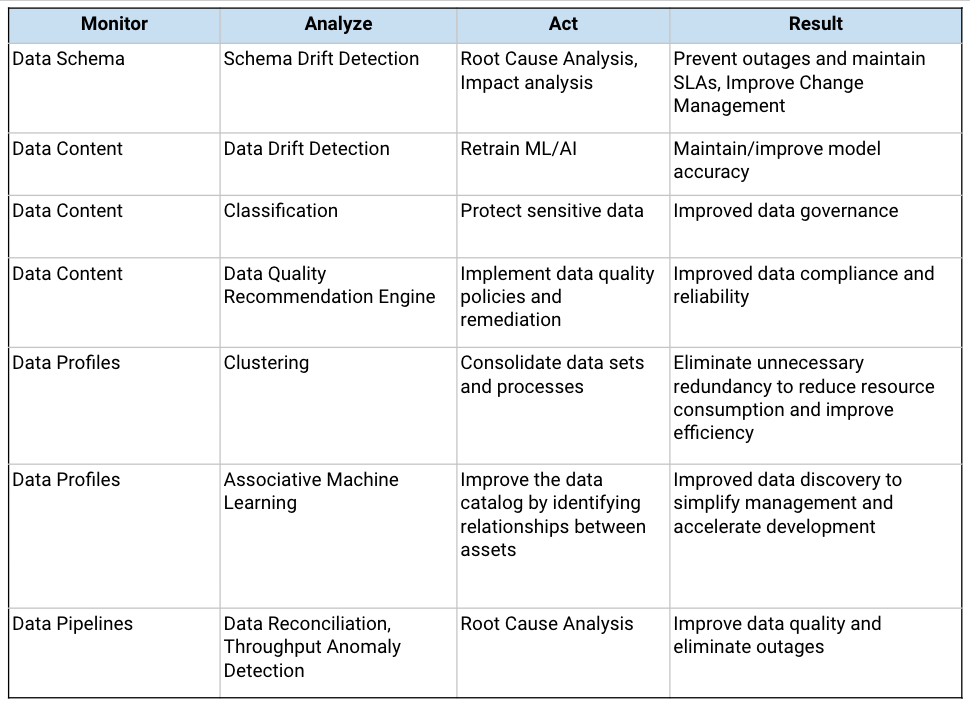
Data Pipeline Management
Data Observability can provide insights for optimizing the design, management, and strategy of data-driven business processes because it makes pipelines easier to manage by surfacing insights into data quality, consistency, performance, and other metrics that are inaccessible using other approaches.
It can enable design-to-cost, forecasting and alignment of business and technical strategies by blending financial analysis capabilities with pipeline telemetry.
Common use cases include:

Summary
As digital transformation initiatives drive more data and analytics use cases, the complexity of data operations increases. Data Observability takes a concept from control theory used to manage complex systems and applies it to Data Operations.
The relatively simple world of traditional data warehousing can be engineered for autonomy, like the automated people mover at an airport. However, you can’t engineer a track for everywhere you want to go. Today’s use of analytics calls for a self-driving car, a vehicle that can observe and react on any road in any weather condition.
Join us for a demo of the Acceldata platform to learn more.