One of the key benefits of digital transformation (DX) is the democratization of data — who has access to it, who can analyze it, and who holds the resulting knowledge for influencing vital processes and decisions inside a modern business?
Democratization, which Gartner declared was one of the top ten technology trends for 2020, is a great thing, especially for data. Until recently, access to vital data was not just unequal — but downright authoritarian. Think of the powerful grip of your naysaying database administrator. Or the monopoly on analytics enjoyed by your BI analyst.
Such harsh regimes existed for a good reason. Databases were massive, mysterious, and mission-critical, which is why DBAs protected them with the zeal of high priests. Creating BI dashboards and reports was difficult and time-consuming, even for highly-trained BI analysts.
That has all changed. Innumerable technologies have arisen that have made storing, processing and analyzing data much easier, safer, and faster. They include data lakes and other cloud-based data repositories, and new application development and analytics tools that allow developers — or any line-of-business employee, really — to generate data models without the skills of a fully-trained BI analyst or data engineer.
Empowering a new generation of analytics “power users” — whether they be citizen developers, citizen data scientists, or self-service analysts — enables the use of analytics, machine learning, and operational AI to seep into every process, workflow, business decision, and customer interaction, including real-time ones.
Enabling Embedded Analytics
When analytics becomes widely embedded into an enterprise, businesses make smarter decisions faster, reduce their risks, and boost revenues.
The first prerequisite to enabling embedded analytics is a powerful but easy-to-use self-service analytics (SSA) platform. But there’s more — much more. As Forbes noted recently, companies that want to successfully empower citizen data scientists in its ranks must provide two other things: high-quality and reliable business data, and a strong data and analytics governance process.
One of the upsides of old-school, tightly-controlled data warehouses was the heavy scrutiny given to data before it was admitted into the data warehouse. Data tended to be well-organized and high-quality. The downsides, of course, were the immense time and cost needed to create and manage data warehouses, which is why today’s agile-minded organizations store data in unstructured data lakes.

However, data lakes can quickly turn into data swamps, filled with low-quality, poorly-managed, and redundant data. That lowers performance, raises storage costs, and leads to inaccurate results. That causes users to lose trust in the data. And citizen data scientists and other power users lack the training to detect, manage, and clean up low-quality data.
Meanwhile, weak data governance can not only result in poorly-organized, low-quality data, it can also create security, legal, and compliance risks for organizations trying to democratize access to data. For instance, enabling self-service analytics can create holes for hackers, or let employees accidentally violate privacy laws.
Moreover, simply making data accessible to new users doesn’t mean they can easily find it. For poorly-governed data tends to be difficult to search. Poor governance also raises costs. Without proper oversight, duplicate data sets tend to pop up, as well as duplicate data pipelines connecting data sets to analytics applications.
Drain Your Data Swamp with Data Observability
Before you can responsibly provide data and self-service analytics applications to your citizen data scientists and analytics power users, you need to transform your data swamp back into a clean data lake in order to lower the business and technical risks for your organization.
Data observability helps organizations like yours to improve both data quality and data governance, enabling you to innovate and transform without worry. Data observability solutions empower data stewards and data engineers to view, manage, and optimize modern hybrid data infrastructures and complicated sprawling data pipelines, lowering your business and technical risks, optimizing performance, and ensuring the lowest cost that meets your access needs.
For instance, in many companies, data quality checks have traditionally been performed during the extract, transfer, load (ETL) phase by data engineers. These engineers are burdened not just with creating the data pipelines, but they then must also manually check that the data quality rules are being followed as the data is loaded, combined and transferred. This is granular, tedious work and a waste of a highly-paid data engineer’s time.
A much more efficient approach is to rely on a data observability solution to watch all data while in rest and in motion. Then if a data quality or data pipeline issue arises, the data observability solution will flag the problem and recommend an automated fix. This immensely boosts the productivity of your data engineers and data stewards, allowing them to release more data sets to their citizen data scientists and accelerate the democratization of data.
In other companies, the task of validating raw data before it’s analyzed has traditionally fallen not to the data engineers, but to the data scientists. That not only distracts data scientists from their core job, but forces them to become experts in an area not necessarily in their job descriptions. And asking your untrained citizen data scientists to validate raw data would be a very tall task, indeed.
Again, a good data observability solution will be able to scan and validate data quality and reliability automatically and enable you to apply automated fixes to most of your problems. These can include data governance issues, such as legal, compliance and security, as well as engineering problems in the pipeline. This dramatically lowers the skill requirements for data scientists, enabling companies to safely deploy analytics to a wide base of employees.
Acceldata: A Force for Data Democracy
As the leading data observability solution on the market, the Acceldata platform can be the biggest enabler of data democracy in your organization. Our automated machine learning goes out and automatically classifies your data assets, clusters similar assets together, and gives related assets the same tags. We also automatically scan your data assets for data quality, and provide accurate, one-click recommendations to fix up to 80 percent of your issues. Your data engineers can set multi-policy rules and schedules around automated scans and triggers, and configure the rules to scan your entire data infrastructure, however distributed and heterogeneous. This ensures data is reliable and provides accurate results for AI, ML and other analytics applications. And this enables your data engineers to focus on work that serves the business rather than daily troubleshooting.

Our next-generation data catalog isn’t just an easy way to find the data you need. It is also imbued with powerful automated data discovery and tagging. That means metadata about your data assets is not just applied when first ingested, but updated continually as your data assets — whether at rest or in motion — change over time. That way you have ongoing visibility into data reliability, including data quality, consistency and drift. Our software also taps into the power of crowdsourcing, allowing employees to annotate, tag and rate data sets.
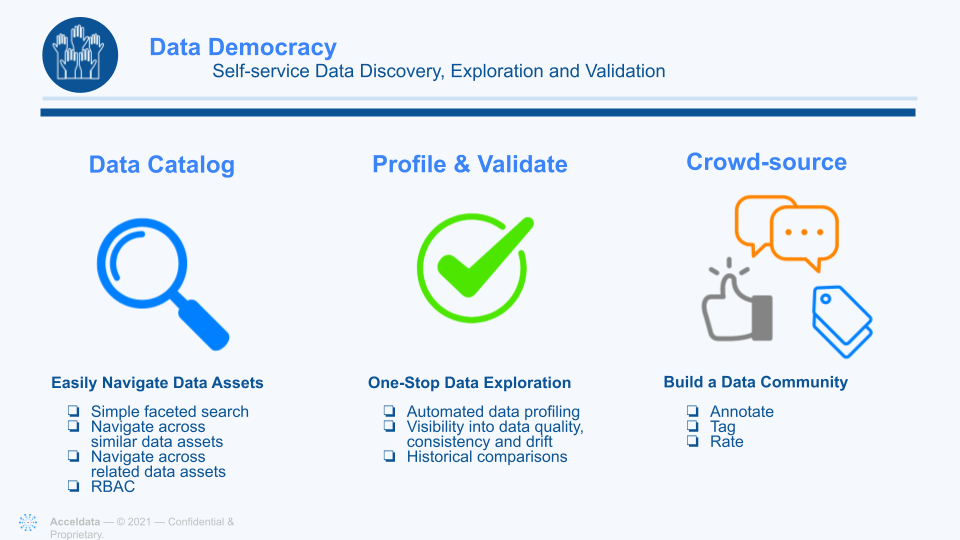
In particular, Acceldata Torch offers powerful features focused on data governance and data reliability. Torch’s data quality rules engine scans for missing data, out of range data, incorrect formats, and more, while its reconciliation features ensures data travels through a data pipeline and arrives as expected. Torch can also detect structural or content changes that lead to schema drift or data drift that can degrade the accuracy of your analytics, AI and ML applications.
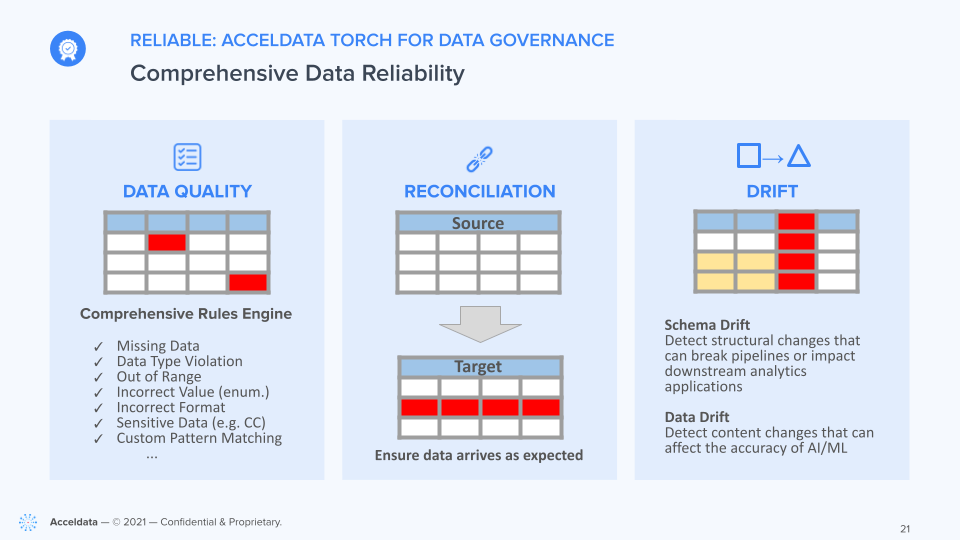
Learn more about Acceldata and how we can enable your organization to democratize data access at www.acceldata.io.