In a recent DBTA webinar, our Acceldata CTO, Ashwin Rajeeva, presented how Acceldata Data Observability can help maintain data quality and integrity in a data fabric architecture.
In this era where data is the lifeblood of business operations, the concept of a data fabric has emerged as a vital architectural approach. Designed to manage and integrate data across diverse systems and environments, a data fabric provides a unified and centralized view of data, fostering seamless data access, integration, and management across an enterprise. However, as enterprises adopt this sophisticated network-based architecture, they encounter several challenges that can impede its effectiveness.
The Essence of a Data Fabric
A data fabric is a single, unified architecture comprising an integrated set of technologies that foster interoperability across various data systems. It democratizes data access at scale, allowing businesses to leverage their data assets more effectively. This network-based approach contrasts with traditional point-to-point connections, enabling more efficient and comprehensive data management.
Gartner defines a data fabric as an advanced data management design aimed at creating flexible, reusable, and often automated data integration across various data sources. It supports both operational and analytical use cases, facilitating seamless data access and integration. By leveraging technologies that enable dynamic data management and interoperability, a data fabric enhances the ability to connect, manage, and utilize data efficiently across an enterprise. This approach simplifies data management by providing a unified architecture that adapts to various deployment environments, ensuring a consistent and comprehensive view of data.
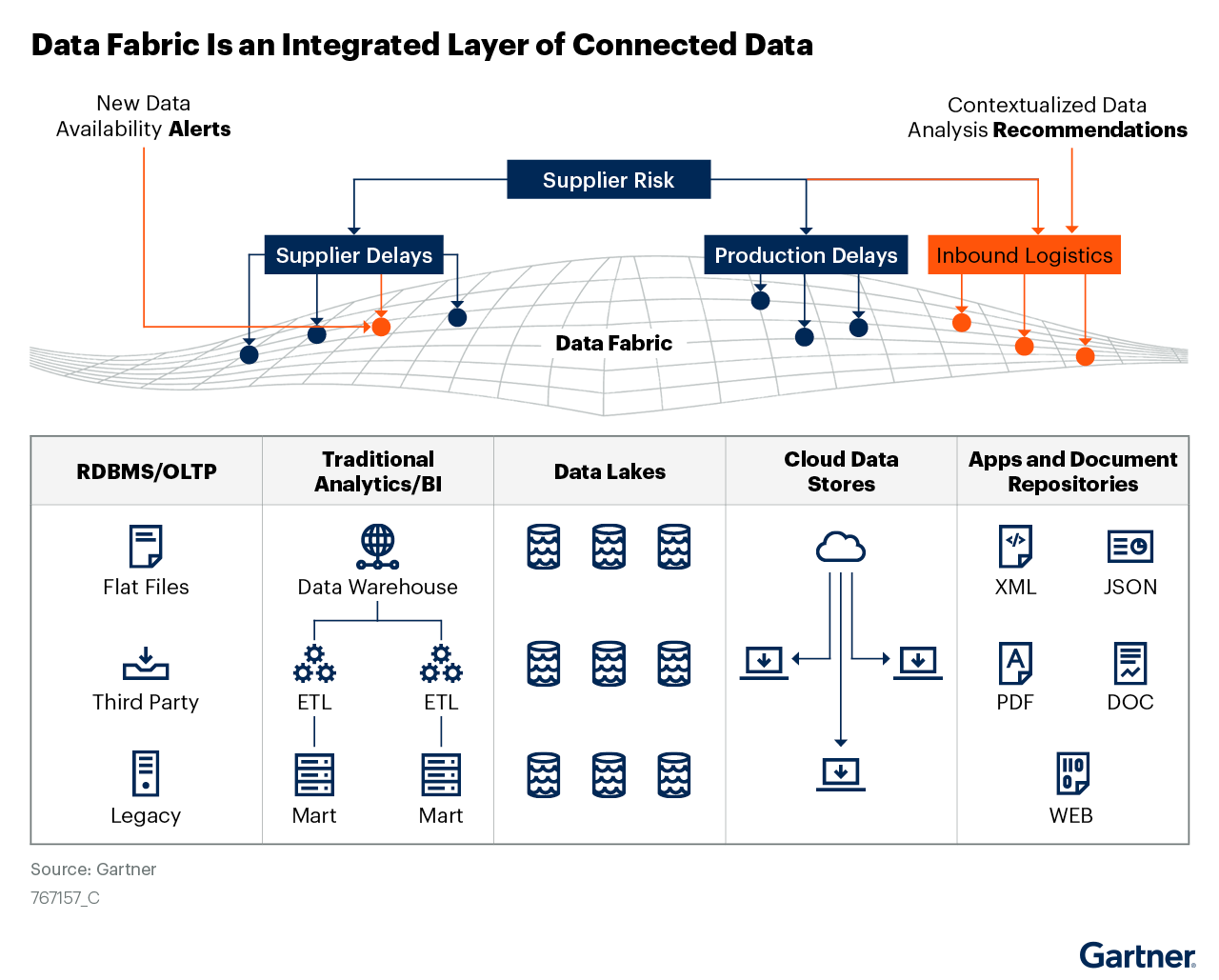
The Six Layers of a Data Fabric
According to Forrester’s “Enterprise Data Fabric Enables DataOps” report, a data fabric is composed of six critical layers:
- Data Management Layer: Ensures data governance and security.
- Data Ingestion Layer: Connects structured and unstructured data across multiple cloud and on-premises systems.
- Data Processing Layer: Refines data, ensuring only relevant data is surfaced for extraction.
- Data Orchestration Layer: Transforms, integrates, and cleanses data, making it usable across the business.
- Data Discovery Layer: Identifies new opportunities to integrate disparate data sources.
- Data Access Layer: Manages data consumption, ensuring proper permissions and compliance with policies.
Enterprise Data Architectures are getting Increasingly Complex
In today's enterprise environment, the complexity of the Enterprise Data & AI Stack introduces significant challenges that directly affect data reliability and quality. With complex data pipelines, a multitude of data sources operating at petabyte scales, and the coexistence of legacy and modern data stacks, maintaining trustworthy data has become increasingly difficult. The emergence of new architectural paradigms such as data fabric further amplifies these complexities, demanding robust solutions to seamlessly integrate and manage data. This complexity underscores the critical need for effective data management to drive innovation, enable informed decision-making, and support successful AI/ML applications.
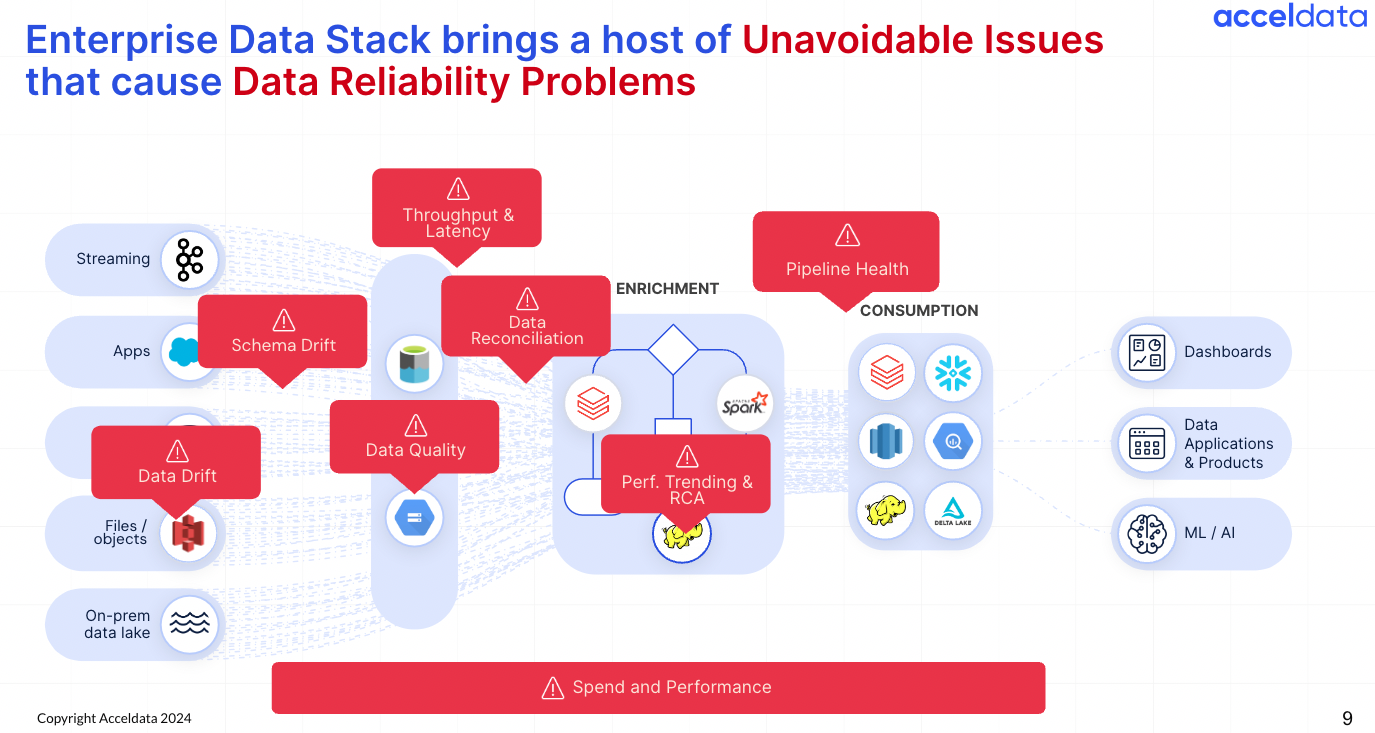
Addressing the Challenges of a Data Fabric Architecture
The data fabric architecture offers significant advantages by enabling a unified and centralized approach to data management across various systems and environments. However, to fully harness its potential, several challenges must be addressed to ensure the architecture functions effectively and efficiently:
- Data Silos: These hinder the seamless flow of information. When data is isolated within different departments or systems, it becomes difficult to create a unified view, impeding holistic analysis and decision-making. Breaking down these silos is essential for achieving comprehensive data integration and fostering collaboration across the enterprise.
- Integration Complexity: Integrating diverse data sources is a time-consuming and complex process. Different systems often have incompatible formats, structures, and protocols, making the integration process technically challenging. Effective integration strategies and tools are required to streamline this process and ensure that data from various sources can be seamlessly combined and utilized.
- Resistance to Change: Organizational inertia can impede the adoption of new technologies. Employees may be reluctant to shift from familiar systems and workflows to new, more complex architectures like data fabric. Overcoming this resistance requires clear communication, training, and demonstrating the tangible benefits of the new approach to gain buy-in from all stakeholders.
- Data Reliability and Trust Issues: These are crucial for effective decision-making and analytics. Inconsistent or poor-quality data can lead to incorrect insights and misguided business decisions. Ensuring data reliability involves implementing stringent data quality checks, validation processes, and maintaining transparency about data lineage and transformations.
- Scalability and Flexibility: Managing increasing data volumes and future growth demands robust solutions. As data continues to grow exponentially, the data fabric must be able to scale efficiently without compromising performance. Flexible architectures that can adapt to changing business needs and technological advancements are essential for sustaining long-term scalability.
- Data Discovery and Metadata Management: These are essential for uncovering and understanding data sources. Effective data discovery processes enable organizations to identify and integrate valuable data assets from across the enterprise. Robust metadata management ensures that data is well-documented, searchable, and easily accessible, facilitating better data governance and utilization.
The Role of Data Observability in a Data Fabric Architecture
Data observability plays a pivotal role in overcoming the challenges associated with a data fabric architecture. It spans all six layers of the data fabric, providing real-time insights into data health, performance, and lineage. This comprehensive visibility helps ensure data quality, pipeline reliability, and infrastructure optimization, which are critical for maintaining the integrity of a data fabric.
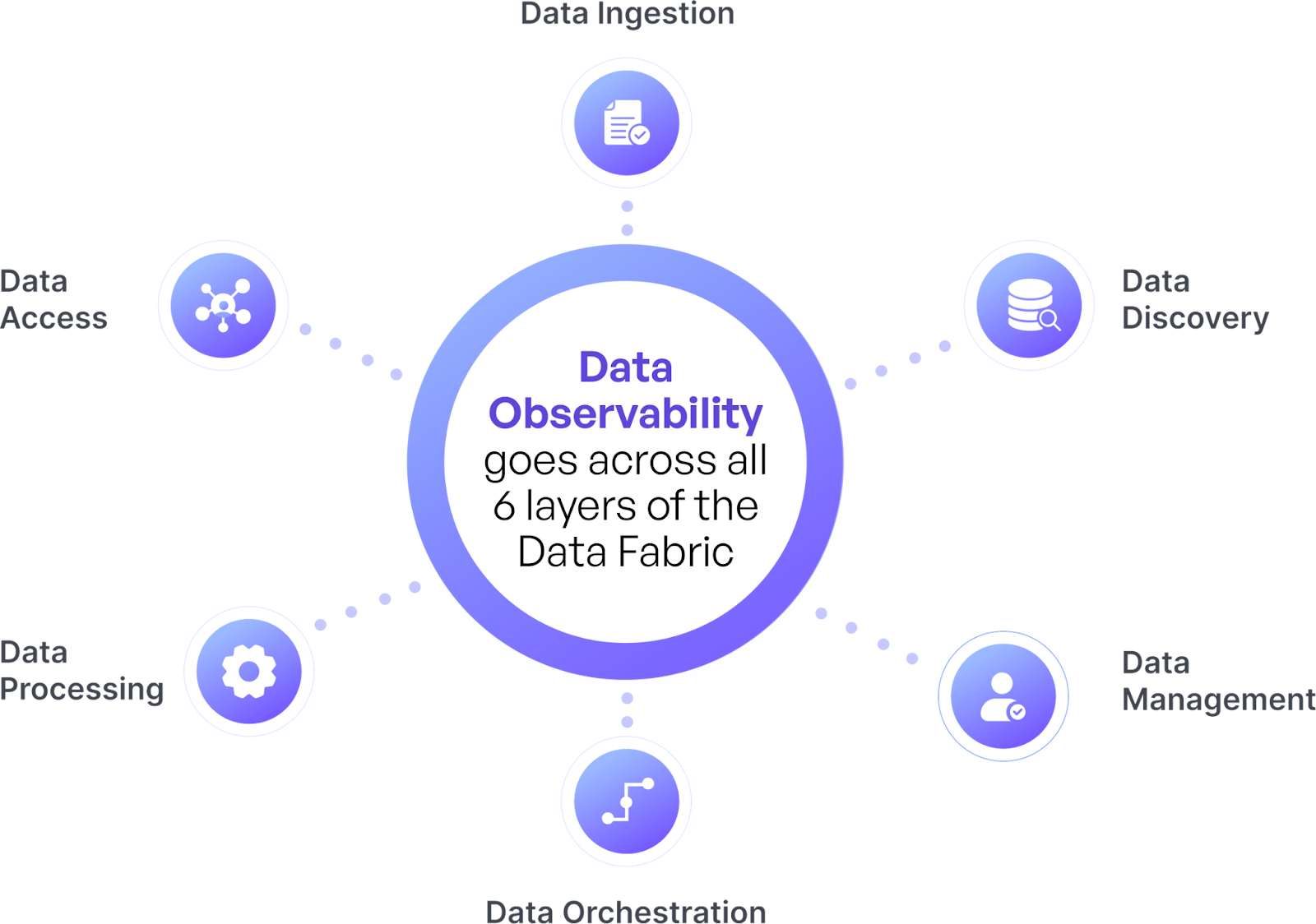
- Data Health Monitoring: Data observability tools continuously monitor the quality and integrity of data as it flows through the various layers of the data fabric. By detecting anomalies, inconsistencies, and errors in real-time, organizations can swiftly address issues before they impact business operations. This proactive approach ensures that data remains accurate, consistent, and reliable.
- Performance Insights: Observability extends beyond mere data quality to encompass the performance of data pipelines and processes. By analyzing metrics such as data throughput, latency, and processing times, organizations can identify bottlenecks and inefficiencies. These insights enable teams to optimize data workflows, ensuring that data moves seamlessly and swiftly across the architecture.
- Data Lineage Tracking: Understanding the journey of data from its source to its destination is crucial for maintaining data trust and compliance. Data observability provides detailed lineage tracking, allowing organizations to trace data transformations, aggregations, and movements. This transparency helps in auditing processes, compliance reporting, and pinpointing the root cause of data issues.
- Pipeline Reliability: Reliable data pipelines are the backbone of a robust data fabric. Data observability tools continuously monitor pipeline health, detecting failures, delays, and performance degradation. By providing early warnings and detailed diagnostics, these tools enable rapid resolution of issues, ensuring that data pipelines operate smoothly and consistently.
- Infrastructure Optimization: Efficient use of infrastructure resources is essential for scalable data management. Data observability offers insights into resource utilization, identifying over- and under-utilized assets. This information helps organizations optimize their infrastructure, reduce costs, and improve overall system performance.
- Unified View and Reporting: A comprehensive observability platform provides a centralized view of the entire data ecosystem. This unified reporting allows stakeholders to access real-time dashboards, generate reports, and gain insights across different layers and components of the data fabric. Such visibility fosters informed decision-making and enhances collaboration across teams.
Incorporating data observability into a data fabric architecture not only addresses existing challenges but also lays the foundation for a more resilient, efficient, and scalable data management system. By ensuring data quality, performance, and reliability, organizations can fully leverage the potential of their data fabric, driving innovation and maintaining a competitive edge in the digital landscape.
Conclusion: Ensuring Reliability of Data in a Data Fabric
In the complex landscape of modern data management, maintaining data quality is increasingly challenging yet indispensable. Poor data reliability can lead to significant business impacts, including loss of productivity, increased costs, and damage to reputation. Data observability provides the tools necessary to monitor, detect, and proactively resolve issues across the data fabric, ensuring that businesses can trust their data and make smarter, data-driven decisions.
Data observability provides data teams with insights about usage, pattern, quality and semantic of data and enables the automation of data suggestions and delivery within a data fabric framework.
By adopting a data fabric enhanced with robust data observability practices, enterprises can achieve a more efficient, reliable, and scalable data management ecosystem, driving innovation and maintaining a competitive edge in the digital age.