Data engineering teams are constantly challenged to ensure that data is of the highest quality to keep data flowing efficiently. To do this in continuously changing data environments, these teams need to learn how to scale data reliability.
Data reliability can often be a moving target as:
- New projects with new data assets and pipelines are completed and rolled out.
- Existing projects have new or changed requirements or grow in scope requiring data asset and popeline change or expansion.
- Previous projects may have been rushed out the door and deployed without the complete level of reliability monitoring/checks.
- Data assets grow in volume and diversity requiring a new level of data reliability monitoring
Add to this the time data engineers are firefighting data incidents and pipeline breakdowns. A recent survey of more than 200 data leaders by Censuswide revealed that:
- 45% have experienced data pipeline failures 11-25 times in the past two years due to data quality or errors that were discovered too late.
- Over half (53%) state that their data teams spend 1-6 days per month addressing data quality issues.
- 58% indicate that dealing with issues related to data quality interferes with other job responsibilities and higher priority projects.
Data teams are constantly scrambling to keep up with the workload, keep projects on schedule, and keep the project backlog low. Consider how data leaders responded when asked, "How many times in the last two years have your data pipelines failed due to data quality or errors that were caught too late?":
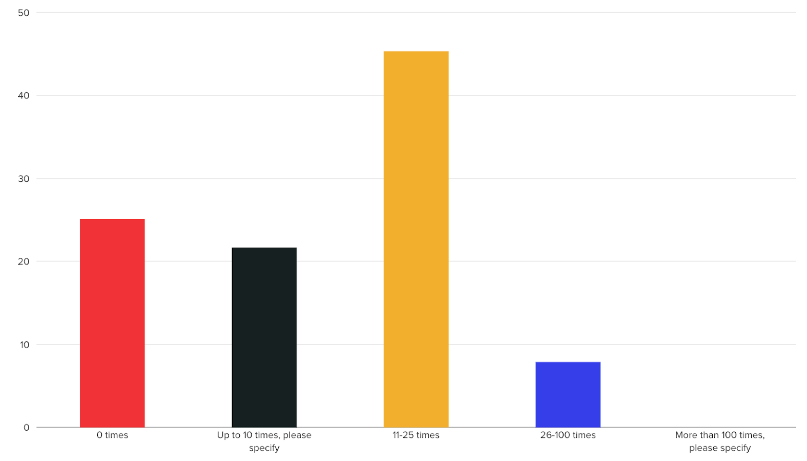
How Modern Data Teams Scale Data Reliability
Today’s third wave of CDOs are challenged to support the business’s ravenous appetite for new data workflows. New forms of data are captured in the hope of being harnessed for new analytics. On top of this, CDOs need to maintain the highest degrees of data governance and security.
So, how do data teams scale out their data reliability efforts?
One way would be to hire more data engineers. But as is constantly noted, data engineers are in high demand, short supply, and require premium compensation. A brute force approach by manually adding more resources would be difficult to achieve and most certainly would send data engineering costs through the roof.
The only way to scale data reliability is through automation, efficiency, and active incident management. Data observability platforms allow data engineering teams to scale their data reliability efforts far more effectively while keeping costs under control. Let’s explore how Acceldata’s Data Observabiity Platform fulfills each of these three areas.
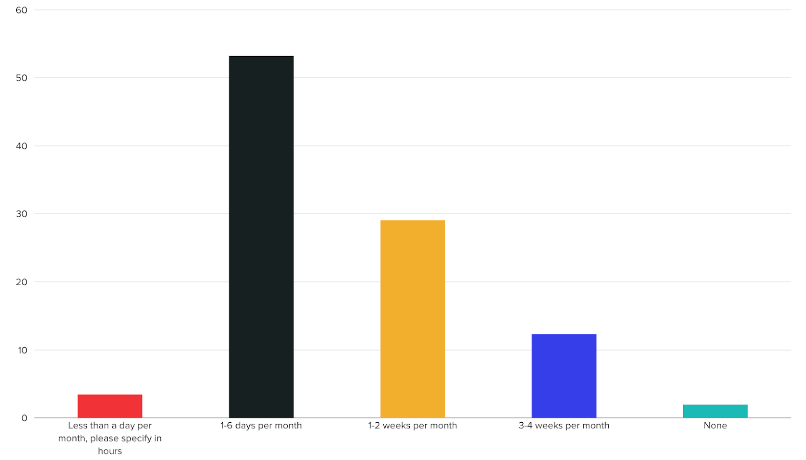
How to Use Automation for Data Reliability
The Acceldata Data Observability Cloud combines artificial intelligence, metadata capture, data profiling, and data lineage to gain insights into the structure and composition of your data assets and pipelines. Using AI, Acceldata:
- Scours the data looking for multiple ways in which it can be checked for quality and reliability issues,
- Makes recommendations to the data team on what rules/policies to use and automates the process of putting the policies in place,
- Automates the process of running the policies and constantly checks the data assets against the rules
The Data Observability Cloud also uses AI to automate more sophisticated policies such as data drift. The Data Observability Cloud constantly learns from the data and automates the process of determining if data is varying from historical patterns and distribution,
Previously, the only way to detect data drift was to manually query the data and either run it against a home-built machine learning model or spot check it visually. Data drift is incredibly important when data pipelines are feeding business AI and ML models as data that has drifted can cause erroneous results in these models and processes they support.
Acceldata also automates the process of data reconciliation used to keep data consistent across various data assets. This automation also eliminates previously highly manual processes for data reconciliation.
Finally, Acceldata uses the data lineage to automate tracking the flow of data between assets during data pipeline runs and the timing. The automated process also correlates performance data from the underlying data sources and infrastructure so data teams can identify the root cause of data incidents, resolve problems, and keep them from happening again.
Through this automation, data teams can scale up their data reliability coverage by running a greater number of policies on data assets and have more sophisticated checks.
How Data Reliability Makes Data Operations More Efficient
Manually putting data quality and reliability policies in place one-by-one for each asset would be a huge drain on data engineering resources. The automation mentioned above helps with team efficiency and productivity, but the automation doesn’t help (a) when custom rules are required, and (b) there are large numbers of data assets that need quality and reliability checks.
To help data teams be more efficient and productive, the Data Observability Cloud offers three critical features:
- No- and low-code tools that speed the process of creating new rules that are not automated,
- Templatable policies that can contain multiple rules which can be applied to data assets in one clean sweep, and
- Bulk application of templated policies which can be applied on any number of data assets at once
In a matter of minutes, a data engineer can create a reusable, templated policy containing multiple rules and apply the policy across many data assets. This helps data teams scale out their data reliability by gaining coverage on more assets.
Incident Management
As evident from the survey results, data teams spend a good deal of their time firefighting data reliability incidents. And often, the data teams are the last ones to know there are data reliability issues, further slowing their time to react.
Acceldata automates the process of running the data reliability policies against the data. It provides a constant vigil on the reliability of data to automatically identify issues. The Data Observability Cloud also provides composable dashboards, automated alerts, assignment of incidents, and problem tracking. Acceldata also provides multi-layer data that correlates metadata, data profiles, quality policy results, data pipeline execution results, and data source and infrastructure performance.
Through the alerts, incident management and deep set of data, data engineers can quickly identify and resolve problems as they occur. This allows data teams to scale their incident response, reduce time to resolution, maintain high data SLAs, and eliminate certain errors from happening again.
Data Observability for Your Data Reliability Strategy
Evn with large data teams, scaling data reliability programs and processes is quite the challenge. With data engineers in short supply and high demand, manual scaling of data reliability is simply not a viable option.
Data observability platforms such as the Acceldata Data Observability Cloud provide the tools and capabilities to:
- Scale up your data reliability via automation,
- Scale out your data reliability via tools that increase team efficiency, and
- Scale data incident management and lower the number of errors.
Learn more about the Acceldata Data Observability Cloud platform and data reliability capabilities and get a personalized demo today.
Photo by Sebastian Pichler on Unsplash