It’s like eBay, but for your company’s data sets.
Leading cloud infrastructure and database providers have created data marketplaces that enable companies not normally in the information business to have a side hustle hawking their internally-generated data to other companies.
Data marketplaces are a great opportunity for any business to maximize the value of their fastest-growing asset. However, there are some emerging best practices that companies need to follow to sell successfully. In this blog, I will:
- Give an overview of the new crop of data marketplaces
- Explain the four key ways they differ from their predecessors
- Detail the four most-common mistakes that newbie companies make when they list their first dataset on a data marketplace
- Show how a multi-dimensional data observability platform can help data sellers overcome those issues
Rocking the Data Casbahs
For anyone in the data industry, news about emerging data marketplaces have been coming fast and furious in the last few years, with a definite acceleration taking place during COVID-19.
They include:
- AWS Data Exchange, which launched in 2019 and today offers more than 3,700 free and paid datasets to companies. Many of the datasets are already formatted for immediate use with Amazon solutions like its DynamoDB transactional database or its Redshift data warehouse.
- Snowflake Data Marketplace, which launched in 2020 to much fanfare and offers more than 1,300 datasets from 300 companies, all ready to query from its namesake cloud data warehouse.
- Oracle Data Marketplace, an outgrowth of the Oracle BlueKai Data Management Platform which the company acquired in 2014. Oracle claims it has the “world’s largest third-party data marketplace” with more than a billion profiles and 30,000 different data attributes.
- The SAP Data Warehouse Cloud Data Marketplace, from the enterprise software giant, launched in 2021.
- Nokia Data Marketplace, a collaboration between the telecom vendor and hosting provider Equinix, also launched in 2021 offering a new twist — payments via Nokia’s private blockchain.
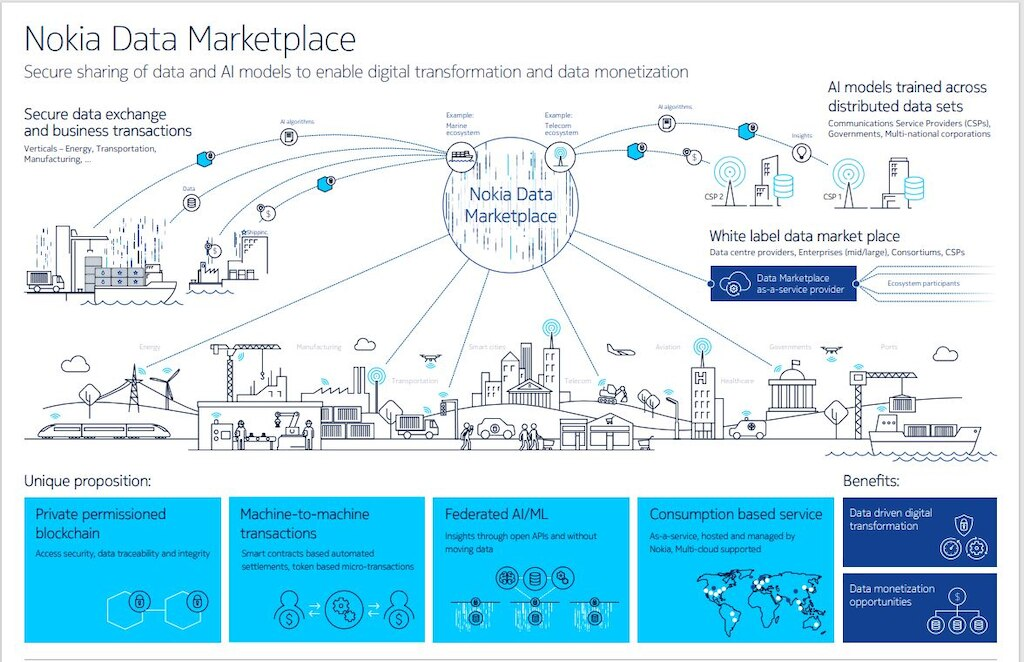
Source: Nokia.com
Also launching in 2021 were two data vendors — one old, and one new — releasing data marketplace-like platforms:
- The Informatica Cloud Data Marketplace from the ETL and data governance pioneer. “Marketplace” is metaphorical, as Informatica’s platform lacks buying and selling features, instead focusing on enabling users within the same company to easily share datasets with other employees.
- Databricks Delta Sharing is an open protocol for secure data sharing between customers, suppliers and partners. Delta Sharing removes the friction of connecting disparate systems with different data formats, security and governance policies. Like Informatica’s offering, it lacks a monetization element. Unlike most of the previously-mentioned offerings, Delta Sharing does not require the use of Databricks’ platform, nor its spin on the cloud data lake, the Delta Lake.
Old Idea — Four New Twists
Data marketplaces are hardly a new thing. Audience data marketplaces and data exchanges selling consumer profiles, and location and contact info to e-commerce companies and Internet advertisers have been around for decades. Audience data was a $22 billion market in the U.S. alone last year.
Traditionally, the sellers in audience data marketplaces have been market research and adtech companies focused on collecting, processing, and delivering ready-for-campaign datasets. And most of these marketplaces — with names such as Adform, Adobe Audience Manager, Lotame, etc. — are tied directly to Demand Side Platform (DSP) or Data Management Platform (DMP) software that is sold to the same e-tailers and marketers buying the data.
So what is different about this new crop of data marketplaces? Four things:
The Buyers. Audience data marketplaces are the stomping grounds of retailers and advertisers looking for demographic and contact info to better target consumers. By contrast, these new data marketplaces cater both to those existing buyers as well as a new breed of users coming from a wide variety of organizations:
- Data scientists looking for datasets to train their ML models and improve their predictive power on a variety of business topics.
- Business intelligence and analytics users who want to combine their company’s proprietary in-house data with external sources, both historic datasets and real-time streaming feeds, in order to perform more sophisticated queries and answer more difficult questions.
- Developers of data applications (also known as data-driven or data-intensive applications) that use an increasing variety of internal and external data sources to deliver rich insights or automate operations.
The Sellers. With the mainstreaming of technologies such as big data, data science, machine learning and AI, today’s companies are not only proficient at using data to drive their businesses, but also boast many data engineering/ops experts capable of curating, preparing and sharing data. They create comprehensive data catalogs and oversee metadata taxonomy systems that enable efficient self-service discovery of data by any employee. Their goal is to dismantle dreaded data silos, democratize access to data, and maximize the value of data through maximum reuse. Those are the skills and mindset that companies need if they want to take the next step and enter an external data marketplace.
The Technology. In the era of data centers, server clusters and proprietary databases, sharing data was clumsy and slow. According to AWS Data Exchange’s general manager, Stephen Orban, even recently many companies were “still literally shipping hard drives to each other” or using 80s-era FTP sites. Selling data simply wasn’t worth the trouble for companies that weren’t 100 percent dedicated to it. In today’s era of cloud data lakes, managed, serverless databases, and quick-build microservices, it is feasible for any company to start selling data — especially when the marketplaces are integrated with their existing database or cloud platforms. These data marketplaces also make it much easier for buyers to ingest, transform, and stay synced with sellers’ data sources. No need for difficult-to-build ETL pipelines. The marketplaces also handle all of the small-but-vital details to make a transaction go through, including tracking (whether usage-based, query-based, compute time, or time-based), invoicing, billing and payments.
The Economic Opportunity. As noted above, audience data is already a massive market worth tens of billions of dollars every year. But as data marketplaces bring mainstream enterprises on board en masse, they will soon dwarf the audience data market, experts say. Accenture, for instance, estimates that by 2030, more than one million organizations worldwide will be selling data assets to the tune of $3.6 trillion.
Benefits Abound
For AWS, Snowflake, Oracle and SAP, running data marketplaces can earn them a tidy sum for every dataset rented or sold. More importantly, data marketplaces add value in the same way that app stores add value to smartphones: driving greater usage of their cloud-based platforms which translates directly into more revenue.
Today, it’s not just the high-profile data-driven companies like the LinkedIns or Netflixes of the world that have data worthy of selling. Every company — from large enterprises to small businesses to startups — generates large amounts of data more valuable than they may suspect.
Your company’s data doesn’t have to fit into one of the big buckets — the $12 billion market for location data, or sectors such as retail, financial services, and healthcare — to be of value, either. Any large-scale set of customer behavior or transactional data, including B2B customers, can be useful. It just needs to be properly formatted, cleansed, anonymized and transformed so that it’s ready for queries.
Whereas companies in the past used to buy pre-packaged market research to insert into static reports, nowadays companies have the skills to analyze external datasets to generate their own original insights or build dashboards for ad hoc data exploration.
For instance, during the early days of COVID-19, economists were measuring the severity of the virus by analyzing daily restaurant reservation trends, and tracking compliance with governmental lockdowns by crunching mobile phone location data . They were also tracking employment levels by analyzing employee hours from a business time scheduling app.
As SAP put it: “Data is the new currency of the digital economy, and data providers are at the heart of it.”
Prepping Your Dataset for Sale: How to Avoid these Four Pitfalls
While data marketplaces make it incredibly easy to get started selling data, that doesn’t mean your company will be effective.
Below are the four most common issues that newbie data sellers need to solve if they want to sell effectively. While most are familiar to any data engineer experienced with creating an internal data catalog or self-service platform, that doesn’t mean they aren’t difficult and time-consuming to solve. Which is where a multi-dimensional data observability platform can come to the rescue.
- Poor Data Discoverability. The most common rookie mistake. Data sets need to be tagged with the right metadata and/or cataloged properly to make it both easy to find and attractive to potential buyers. It’s like ensuring your webpage has the right keywords so it will have strong SEO. A data observability platform is proficient at automating the process of cataloging datasets, finding missing ones and eliminating duplicate datasets. It is also good at applying the right metadata to ensure easy data discovery. Moreover, that cataloging and metadata tagging can’t happen just once when the data is ingested. Datasets evolve and drift over time or simply get stale. These changes over time must be continually updated in the metadata and data catalogs. The right data observability platform automatically re-scans data at regular intervals or when triggered by changes it detects in the dataset. That ensures good data discoverability over the long haul.
- Untrusted Data. Another common mistake with companies new to selling on a data marketplace is failing to clean, format, and otherwise prepare their data as professionally as the Dun & Bradstreets, Equifaxes, and other information brokers of the world. And even if the dataset is ready for queries, they need to document and validate it to win naturally-skeptical customers. Data observability tools offer features that not only improve data reliability but also validate it, creating trust in data. First, they detect the freshness, accuracy and frequency of data. Then, applying these defined rules, they can certify a dataset as ‘gold’ if it meets desired thresholds. This information greatly increases trust in data by potential buyers.
- Managing Schema Changes. To keep data as updated as possible, data marketplaces such as Snowflake and AWS promise to share live data between seller and buyer rather than copy it. Or they will trigger data syncs when it detects changes in the source dataset, similar to a database’s Change Data Capture (CDC) process. That’s all well and good. However, that is not enough if/when you the data producer makes a schema change to your dataset. The data in the records may not have changed, but crucially the table structure has. You have added new columns, or changed a column label or data type. Those schema changes are disruptive to your internal data pipelines and users. But that disruption is magnified when it comes to marketplace buyers of your dataset, due to the virtual distances between your respective organizations. Using a data observability platform, a data producer or data consumer can immediately detect if a dataset’s schema has changed or drifted by comparing old and new versions. They can take steps right away to minimize the potential impact, such as erroneous reports or automated operations going haywire.
- Building Knowledge Graphs of Data Producers, Curators, and Consumers. Creating data catalogs and applying taxonomy metadata certainly makes data sets more discoverable to potential buyers. However, you can further improve data discoverability by tracking and labeling the dataset’s lineage from original source until its uploading into the data marketplace. Knowing how data has flowed, been used, and transformed is valuable metadata that can help a potential buyer determine its relevance. It can also be used by a data marketplace when it suggests relevant datasets to users, similar to the way Spotify analyzes your profile, existing playlists and liked songs to suggest other songs you might like. For example, a weather dataset may be useful for retail stores to properly size how much inventory to keep. But if that weather dataset has also been used by warehouses, wholesalers and others within a local retail supply chain, or even by a local government agency looking to prepare for catastrophic weather, that is useful information. And it could help boost interest among dataset shoppers with similar profiles, leading to more sales. Data observability platforms today can already capture internal data lineage information and create first-generation knowledge graphs from that. The next step will be for data observability platforms to capture usage data as well as social likes and recommendations from the data marketplace where your datasets are being sold. I expect that to be coming soon.
Our Solution
Acceldata’s multidimensional data observability platform can help companies like yours more effectively monetize your datasets through data marketplaces.
In particular, our Acceldata Torch module provides a full suite of capabilities that automatically ensure ongoing data quality and reliability across the entire data pipeline. Torch also automates the validation of your data reliability by classifying, cataloging, and managing business rules for data at rest and in motion.
While improving data quality and reliability is nobody’s idea of fun, it’s a task that can be greatly eased and automated with Acceldata Torch. It can turn your data engineers into 10x superstars. And it helps your team more easily address the four pitfalls of newcomers to data marketplaces.
Photo by CHUTTERSNAP on Unsplash