How Top Enterprises Ensure Data Trust at Scale
Introduction: Why Flexibility Is Your Data Superpower
In today's multi-cloud, complex data landscape, unreliable data isn’t just an inconvenience—it’s a multi-trillion-dollar problem. A 2023 IBM report estimates that poor data quality costs U.S. businesses $3.1 trillion annually, while an IDC study (2024) reveals that only 44% of users trust their data for analytics.
The solution? Data observability that adapts to your workloads—whether processing in-place for moderately sized datasets or scaling for massive, high-volume transformations. That’s where PushDown and ScaleOut (Spark) execution modes come in, now supercharged with Acceldata’s Agentic Data Management (ADM) to unify, think, and act on your data.
PushDown Execution: Moderate Datasets and Simpler Data Quality Requirements
What It Is
PushDown execution runs observability tasks—such as quality checks, schema drift detection, and freshness monitoring—natively within platforms like Snowflake or Google BigQuery, leveraging built-in compute power. As the name suggests, it pushes operations down into the source data system, now enhanced by ADM’s AI agents for autonomous data reliability
Who Benefits Most
- Regulated Industries – A healthcare giant used PushDown in Snowflake to meet HIPAA compliance while profiling patient data securely in-platform.
- Cost-Savvy Teams – Enterprises with moderate data volumes save big by avoiding external compute.
Supported Platforms
ScaleOut (Spark) Execution: Larger Datasets and Advanced Computational Needs
What It Is
ScaleOut execution harnesses Spark’s distributed computing to handle high-volume, cross-platform workloads—like anomaly detection, reconciliation, and deep analytics. As the name suggests, it pulls data into Acceldata’s Spark cluster within your environment, powered by ADM’s xLake Reasoning Engine for AI-driven scalability.
Who Benefits Most
- Big Data Players – A retailer reconciled supply chain data across AWS and Databricks, spotting inconsistencies faster than traditional batch processes.
- AI Innovators – Teams leveraged ScaleOut’s scalability and compute power for ML-driven observability.
Supported Platforms
PushDown vs. ScaleOut: Your Decision Cheat Sheet
Pushdown Execution Mode:
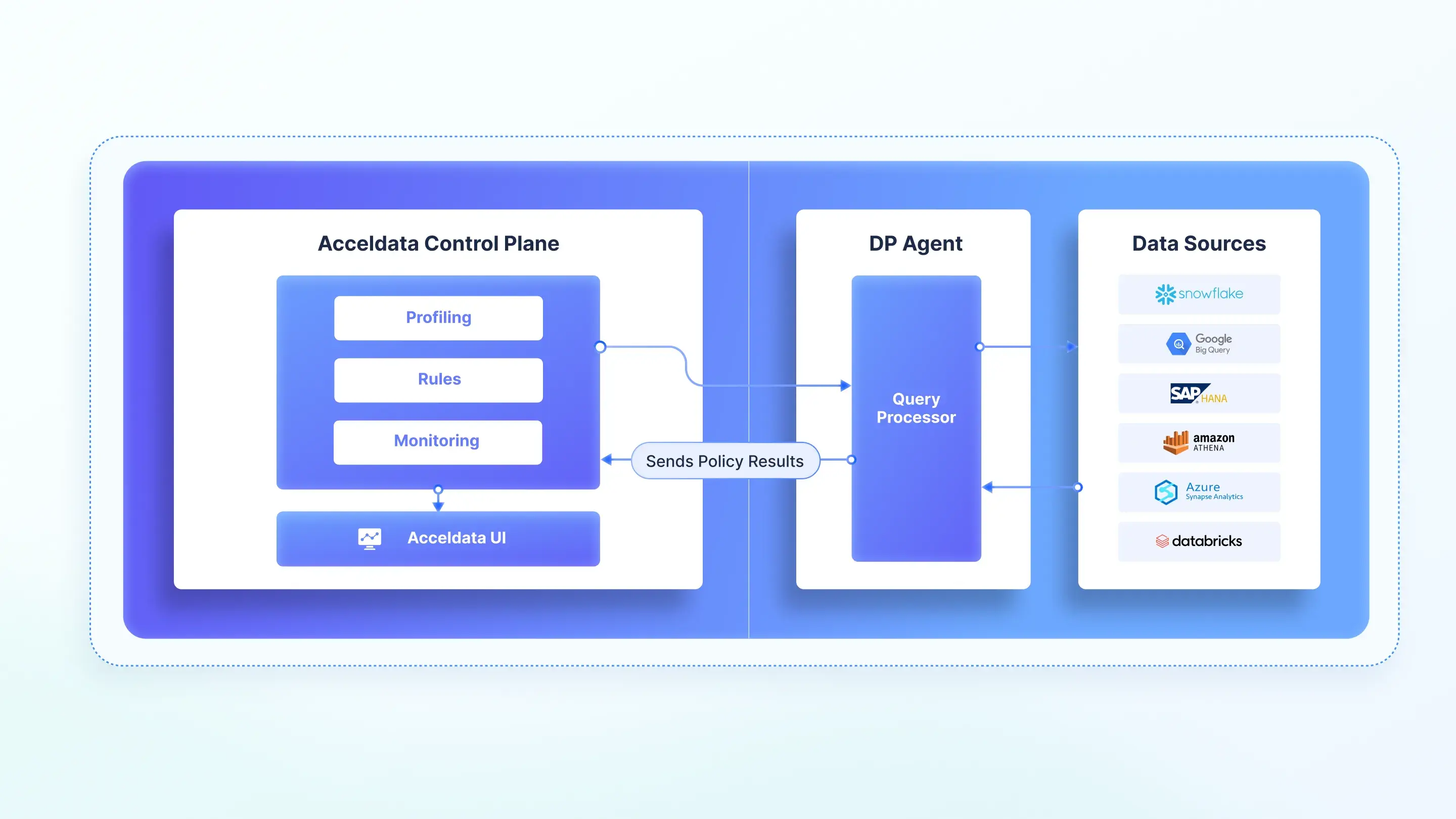
ScaleOut Execution Mode:

Why Leading Enterprises Choose Acceldata
Acceldata pioneered PushDown and ScaleOut execution in data observability, now enhanced by Agentic Data Management—empowering enterprises to optimize every workload dynamically.
- Switch execution modes per workload—no lock-in
- Monitor and improve cloud data quality with ADM’s autonomous agents
- Balance cost and performance with intelligent automation
Real-World Impact
A pharmaceutical leader slashed costs and ensured GDPR compliance by using PushDown for in-platform checks in Snowflake and ScaleOut for AWS transformations, handling petabytes of patient data without trust gaps.
Your Next Step: Future-Proof Your Data Observability Strategy
Data chaos isn’t inevitable. With flexible PushDown and ScaleOut execution modes, powered by Agentic Data Management, enterprises cut costs, ensure compliance, and scale confidently for an AI-first world.
Schedule a Demo to explore how Acceldata’s dual execution and ADM transform your data strategy.