The world’s largest and best-known brands rely on data to fuel their business growth. By identifying customer trends, personalizing customer needs, and achieving internal operational excellence, companies like Netflix, JCPenney, Airbnb, and numerous other trailblazers surpass their competitors and dominate their respective markets.
While there are a myriad of concerns that data teams are concerned with, there are five essential issues they all must address in order to maximize the efficacy of their data investments. Addressing these use cases can enhance cost optimization and elevate decision-making at every level. Below, we delve into these five key use cases, offering insights from top organizations on how they leverage data observability to confront them head-on.
How Data Observability Became the Foundation for Business Excellence

Data observability has become a pivotal ingredient for data teams that want to successfully deploy their resources for better organizational decision-making and improve operational ROI. At its core, data observability refers to an organization’s ability to fully understand and manage the health, quality, and performance of data. This comprehensive approach is not just a technical necessity but a strategic asset, vital for modern businesses to thrive in a competitive environment.
Organizations can make more informed decisions by ensuring data accuracy, data reliability, and timely insights, and potentially drive significant cost savings through cost optimization. From preventing costly downtime to optimizing operational efficiencies, this blog will explore five critical use cases where leveraging data observability can be a game-changer in preserving and enhancing your business's financial health.
Use Case #1: Better Control Over Financial Management
How to Proactively Identify and Address Budget and Finance Issues
The importance of data quality in a business's financial reporting and analysis cannot be overstated. Accurate and reliable data is crucial for preparing financial statements. Poor data quality can lead to errors in balance sheets, income statements, and cash flow statements, vital for internal decision-making and external reporting. Businesses are often subject to stringent regulatory standards regarding financial reporting (like GAAP or IFRS). High-quality data ensures compliance with these regulations, reducing the risk of legal penalties, fines, or reputational damage.
A notable example of a company that leverages data observability to enhance the quality of its financial reporting and analysis, ultimately leading to cost savings, is General Electric (GE). GE, a multinational conglomerate with diverse operations in areas like aviation, healthcare, and energy, faces the complex task of managing vast amounts of financial data across its various business units.
GE has integrated sophisticated data observability tools into its financial systems, enabling the company to monitor and analyze fiscal data from multiple sources in real-time. Their observability tooling promotes high-quality data that allows GE to generate more accurate financial reports. This accuracy is crucial for internal decision-making, external reporting, and compliance with regulatory standards such as the Sarbanes-Oxley Act.
GE also uses this information to proactively identify and address issues such as budget overruns or unexpected expense spikes. This dynamic approach to financial management helps avoid costly financial missteps like budget overruns, cash flow issues, inaccurate reporting, or even missed revenue opportunities.
Use Case #2: Reducing the Cost Implications of Bad Data With Early Issue Detection
How to “Shift Left” Your Data Reliability Efforts
A comprehensive data observability platform enables businesses to detect anomalies and errors early in their data lifecycle. This capability is essential for maintaining data integrity and operational efficiency. A robust observability solution will continuously monitor data across various stages and systems, thus tracking data flow from ingestion to storage and processing. When coupled with real-time alerts and notifications, real-time monitoring can detect deviations from standard data patterns before the consumer is impacted.
Many data observability platforms leverage advanced analytics and machine learning algorithms to predict and identify anomalies. These algorithms are trained on historical data, enabling them to recognize patterns and deviations that might not be apparent to human observers. This predictive capability is particularly valuable for the early detection of complex issues that could lead to significant problems if left unaddressed.
To tackle issues like data drift and schema drift, a shift-left strategy entails implementing early detection mechanisms at the data landing zone. This involves embedding adaptive machine learning models that continuously learn from historical data, allowing them to adapt to subtle shifts in data patterns and schema alterations. Additionally, automated schema validation checks should be integrated into the data landing zone to ensure incoming data conforms to predefined structures, minimizing the chances of schema drift.
Managing data reconciliation challenges in a shift-left approach involves extending real-time monitoring capabilities to include data reconciliation processes. By monitoring data consistency across different zones in real-time, organizations can quickly identify and rectify discrepancies, maintaining high-quality data across the entire pipeline. Implementing a robust historical data tracking mechanism within the observability platform enables data teams to trace changes and reconcile discrepancies by comparing current data states with historical records.
To foster a shift-left culture in data reliability, organizations should encourage a proactive approach to reliability checks at the data landing zone. This cultural shift ensures that potential issues are addressed closer to the source, minimizing the impact on downstream processes. Furthermore, promoting collaboration between data engineers, analysts, and data scientists facilitates the implementation of holistic solutions that encompass both technical and analytical aspects of data quality. Leveraging automation is crucial for enabling swift remediation of issues detected in the data landing zone, ensuring that corrective actions are taken promptly and reducing the likelihood of data anomalies propagating through the pipeline.
Consider the image below where data pipelines flow data from left to right from sources into the data landing zone, transformation zone, and consumption zone. Where data was once only checked in the consumption zone, today’s best practices call for data teams to shift left their data reliability checks into the data landing zone.

How data reliability can shift-left
One notable example of a company that saved money by detecting data errors earlier in its pipeline is Airbnb. As a data-centric organization, Airbnb relies heavily on data to inform its business decisions, optimize its platform, and enhance user experiences. However, managing vast amounts of data comes with significant challenges, including the risk of data errors that can have far-reaching implications.
Airbnb faced data quality and reliability issues, affecting their decision-making processes and customer experiences. To address this, they developed and implemented an internal data observability tool called "Minerva". This system was designed to ensure the accuracy and consistency of their metrics across the organization. Minerva automated the data quality monitoring process, which significantly reduced the manual effort and operational costs associated with data quality checks. This automation allowed data engineers and scientists to focus on more value-added activities rather than spending time identifying and fixing data issues.
Use Case #3: Using Data For Improved Decision Making
Once Data Hits the Landing Zone, Data Observability Can Identity the Needed Information to Help Business and Data Team Goals Intersect
A strategic executive makes decisions informed by experts and backed by data. Data observability ensures that the data used for these decisions is of high quality and accuracy; after all, headlines have repeatedly proven that bad data can be extremely costly.
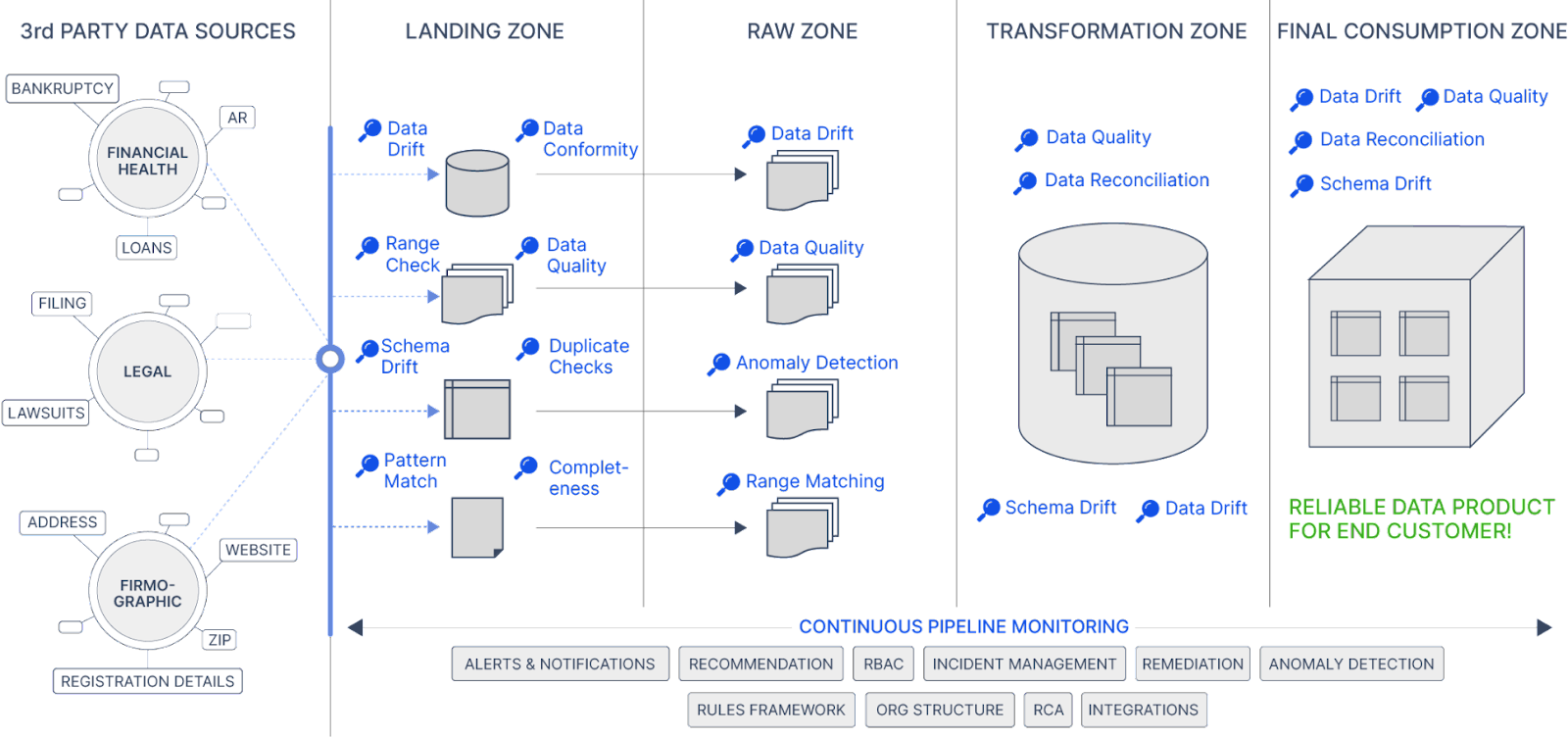
The right data observability approach helps achieve higher data ROI by enabling better decision-making
When data-driven decision-making processes reach the landing zone in a data pipeline, several key improvements occur that enhance the overall effectiveness and reliability of decision-making within an organization. They include:
- Data Quality Reliability: The landing zone is the initial point where raw data enters the pipeline. Implementing data quality checks at this stage ensures that the incoming data is accurate, complete, and conforms to predefined standards. This, in turn, enhances the reliability of the data used for decision-making, reducing the risk of errors and inaccuracies downstream.
- Early Anomaly Detection: By incorporating real-time monitoring and anomaly detection mechanisms at the landing zone, organizations can identify deviations from expected data patterns as soon as the data enters the pipeline. Early anomaly detection allows for timely intervention and correction, preventing the propagation of inaccurate information through subsequent stages of the data lifecycle.
- Reduced Latency in Insights: Processing and preparing data at the landing zone contribute to reduced latency in delivering insights. Decision-makers can access more up-to-date information promptly, enabling them to respond quickly to changing conditions and make decisions based on the most recent and relevant data.
- Streamlined Decision Workflow: The landing zone serves as the foundation for organizing and structuring incoming data. When this foundational layer is well-organized and optimized, decision-makers experience a more streamlined workflow. This allows them to focus on analysis and interpretation rather than grappling with data quality issues, resulting in more efficient and effective decision-making processes.
- Enhanced Scalability: A well-designed landing zone supports scalability, allowing organizations to handle increasing volumes of data without sacrificing performance. This scalability ensures that decision-makers can access and analyze a growing amount of information, supporting strategic planning and decision-making at scale.
Optimizing data-driven decision-making at the landing zone involves ensuring data quality, implementing early detection mechanisms, enforcing governance, facilitating efficient integration, reducing latency, streamlining workflows, enhancing scalability, and supporting advanced analytics. By addressing these aspects at the outset of the data pipeline, organizations can significantly improve the reliability and impact of their data-driven decision-making processes.
Without the right level of data observability, these issues cannot adequately be addressed, and that prevents organizations from using data effectively to make informed decisions. One well-known example of a company suffering significant losses due to decisions made on bad data is the case of JCPenney in the early 2010s. The company, a prominent American retailer, hired Ron Johnson as its CEO in 2011. Johnson, who was previously successful at Apple, attempted to drastically overhaul JCPenney's retail strategy based on assumptions and data that did not align well with the company's core customer base.
Johnson decided to eliminate sales and coupons, a strategy he believed would simplify the shopping experience and attract a more upscale customer base. This decision was partly based on data and insights that worked well in other retail environments, like Apple but failed to consider the unique aspects of JCPenney's market position and customer expectations. The company had not created the right integration of data sources to deliver meaningful insights about customer behavior and predilections.
The company relied, therefore, on misinterpreted and poorly applied data, which led to disastrous brand and economic outcomes for the company. JCPenney's core customers, accustomed to and attracted by regular sales and discounts, felt alienated. The company saw a significant drop in customer traffic, leading to a steep sales decline. In 2012, JCPenney reported a net loss of $985 million, and its revenue dropped by nearly 25% that year.
This example highlights the importance of relying on data for decision-making and ensuring that the data is relevant, accurately interpreted, and applicable to the specific context of the business. It also underscores the need to deeply understand a company's customer base and market positioning when making strategic decisions, all information for which accurate data is essential.
Use Case #4: Predictive Maintenance Eliminates Data Failures
How You Can Make Your Data Work For You
In addition to monitoring data quality, data observability tools provide real-time data systems monitoring. By continuously tracking the performance and health of various components (like servers, databases, and network devices), data observability platforms can identify performance issues before or as soon as they occur. This immediate detection allows for prompt responses, preventing minor issues from escalating into downtime.
Downtime can be incredibly costly for businesses in terms of direct financial losses and damage to reputation. Predictive maintenance enabled by data observability helps schedule maintenance activities like updates before a failure occurs. Tracking resource optimization can also empower data teams to understand usage patterns and make more informed decisions about where to allocate their data resources and spending. This means they can avoid over-investing in underutilized resources or under-investing in areas requiring more attention, leading to more efficient use of capital and operational expenses.
As a leading streaming service with millions of users worldwide, Netflix relies heavily on its vast and complex data infrastructure to deliver uninterrupted, high-quality service. Hence, it’s no surprise the company’s approach to data observability is comprehensive and proactive. They implemented a robust monitoring and analytics system that constantly tracks the performance of their servers, databases, and network devices. This system is designed to detect any anomalies, performance degradation, or potential failures in real-time. While this may sound expensive, Netflix reports “high ROI” on its custom observability dashboards, thanks to increased cost visibility.
By employing advanced data analytics and machine learning algorithms, Netflix's observability framework can predict potential issues before they occur. For instance, if the system detects an unusual pattern in server response times or a spike in error rates, it automatically flags these issues. This predictive insight allows Netflix's engineering team to perform maintenance activities during off-peak hours, avoiding or significantly reducing service disruptions during peak usage times.
Use Case #5: Enhance the Customer Experience
Knowing More Means You Can Deliver More (and Better) Services
Data observability plays a pivotal role in improving various aspects of its data-driven decision-making processes, particularly when it comes to enhancing user experience, personalization, and customer retention. More than anything else, it can provide the most comprehensive utility of your data investment by extending beyond system monitoring to encompass vast sets of customer interaction data. This holistic monitoring approach ensures that not only the technical aspects of the platform are observed but also the customer-centric data, such as viewing habits, preferences, search patterns, and feedback.
Additionally, through the analysis of customer interaction data, companies like Netflix can gain in-depth insights into customer behavior and preferences. This includes understanding what content users are watching, how they search for content, and their reactions through feedback. These insights provide a comprehensive view of user engagement, allowing Netflix to adapt and refine its services based on actual user behavior.
Netflix's data observability extends beyond just monitoring its systems; it also encompasses analyzing vast customer interaction data. This includes viewing habits, preferences, search patterns, and feedback. By observing and analyzing this data, Netflix gains deep insights into customer behavior and preferences, allowing them to tailor their services and content offerings more effectively.
One of the direct applications of these insights is in the personalization of content and recommendations. Netflix uses data analytics to recommend movies and shows that align with individual users' interests. This personalization enhances user engagement, as customers are more likely to find content they enjoy, leading to a more satisfying viewing experience. It’s no secret that happy and satisfied customers are less likely to switch to competitors. By providing a personalized and reliable service, Netflix ensures high customer retention rates. Retaining existing customers is generally more cost-effective than acquiring new ones, as the latter involves marketing and promotional expenses.
How Data Observability Can Work For Your Environment
Leveraging data observability is not just a technological upgrade, but a strategic necessity for businesses aiming to optimize costs and enhance operational efficiency. Data observability empowers businesses to make proactive decisions, from predictive infrastructure maintenance to fine-tuning financial strategies by providing real-time insights into data health, performance, and usage patterns. It enables companies to avoid costly missteps such as budget overruns, inefficient resource allocation, and compliance issues. Implementing data observability is a smart investment, turning data into a powerful tool for cost management and driving sustainable, long-term growth. By embracing this approach, businesses can save money and gain a competitive edge in today’s data-driven world.