Despite the challenges brought about by the COVID-19 outbreak and global economic fluctuations, the global retail industry grew to over $4 trillion in 2020, a 27% jump compared to 2019.
The advent of eCommerce and the rise of digital-first stores contributed to the retail boom, where NASDAQ predicts that 95% of all future retail purchases will be online. Prior to the arrival of online retail, data was amassed primarily through customer surveys and forms. Today, technology has helped broaden that horizon by accumulating finer details, which aids in creating more detailed customer profiles.
With real-time access to a plethora of information surrounding purchase patterns, scrolling behavior, final-mile cart abandonment, geo-tagging, SKU (Stock Keeping Unit) sales volumes, and website bounce-off rates, digital and physical retailers are able to target the right audience with the most befitting campaigns, content, and offers. To drive sales, retailers now rely more heavily on big data analytics and AI-based tools.
However, apart from fine-tuning campaign performance and enhancing marketing initiatives, retailers are also turning towards data-led intelligence to improve backend operations, inventory management, and financial governance. Unless they can rely on reliable, high quality data, retailers today risk losing more than just customers.
Data in the Age of Phygital Retail
With the help of a robust customer data profile, retailers have managed to capitalize on the digital wave. As the number of smartphone devices increases around the world, businesses can monitor content trends according to geographic locations and penetrate age-wise demographics to deliver advertorial content that converts.
However, retailers also believe in the principle of, “Money saved is money earned,” forcing them to utilize different forms of data to reduce costs and improve operational performance.
1. Demand Prediction & Inventory Management
Advanced algorithms and AI-based tools have given retailers the power to analyze purchase trends and seasonal requirements to forecast demand. Products that could lead to a potential spike in demand are stocked up, thereby helping retailers minimize storage costs and handling fees.
2. Price Optimization
Though the concept of “dynamic pricing” isn’t unique to just retailers, online and physical store owners have conducted studies to learn how dynamic pricing is carried out. Through this, retailers have learned that prices tend to drop as demand for a product wears out, and this dip in prices directly leads to a sudden spike in demand for the product. Predictive analytics has helped retailers track the surge and dip in demand, which is why global juggernaut Walmart tracks millions of transactions in real time via a private cloud.
3. Customer Retention
In a study by Bain & Company, it was observed that increasing your overall customer retention by just 5% can boost profits between 25 - 90% because the success rate of selling to an existing customer is much higher. Hence, retailers are spending less on customer acquisition when they can apply innovative ways to keep those customers repeatedly coming back. Data analytics helps businesses engage with customers across multiple platforms, and chart those who are potential life-long customers.
4. Determining Outlet Location
A brand outlet in the right place might be a potential goldmine, but having it in the wrong location can turn it into a landmine. In the real estate sector, there are potentially lucrative locations that turn into metaphorical “dead zones,” where footfall is low and individuals with disposable income do not frequent. In such a scenario, a store can find it difficult to thrive. Analytics helps plot the reason and recommend the right pathway. For example, if parking is inconvenient, there’s a possibility only eateries and coffee shops might survive in that location.
How Data Observability Improves Phygital Retail Operations
While the availability of customer data can work wonders for retail service providers, the quality and integrity of the data play a critical role. Most retailers struggle with this very step, as the data flowing into data warehouses can be disparate and often erroneous. Other challenges include:
- Accuracy of the data, where the information being collected and stored is irrelevant to the insight needed.
- Spreadsheet chaos, where the traditional method of data storage on excel spreadsheets isn’t scalable for Big Data applications.
- Data validation, where the extraction, movement, and verification of data is done manually - which is prone to errors.
- Data compliance, where the possibility of data leaks and hacks can land retailers in a soup due to customer data compliance guidelines (like GDPR in the EU).
Data observability goes above and beyond just routine monitoring. It ensures teams are on top of breakdowns, and manages data across four layers: Users, Compute, Pipeline, and Reliability.
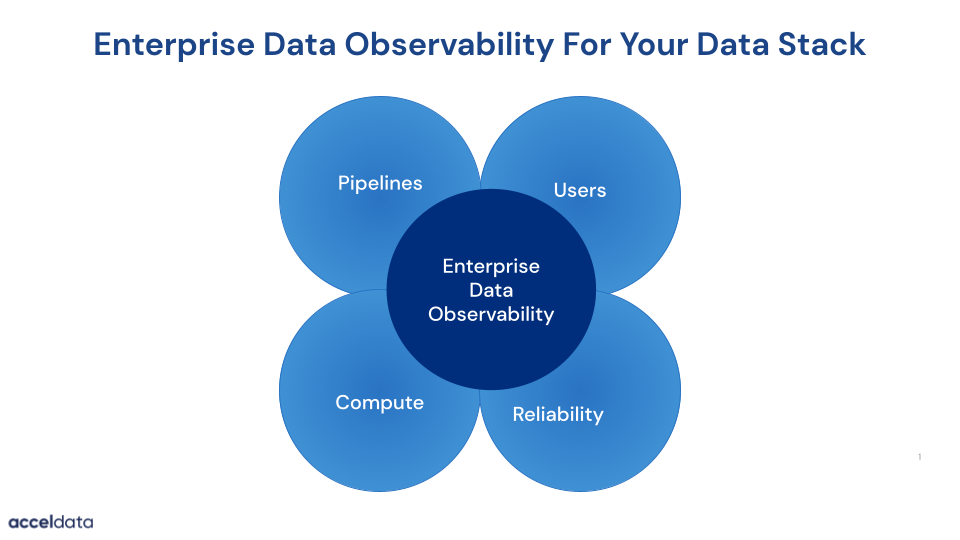
What this means for retail service providers is:
✔ Data flowing across diverse channels and pipelines are monitored in real-time, alerting users in the case of potential breakdowns.
✔ Analytical tool and platform expenditures are tracked and validated.
✔ Data reconciliation and schema drift issues are solved to combat data errors.
✔ A 360-degree view into the quality of data that is required across the organizational infrastructure (such as Data Engineers, Architects, Analysts, CDOs, etc.).
Acceldata is the market leader in enterprise data observability. With Acceldata’s multi-layered data observability solution, enterprises gain comprehensive insights into their data stack to improve data quality, pipeline reliability, compute performance, and spend efficiency.
Acceldata's solutions have been embraced by global enterprises, such as Oracle, PubMatic, PhonePe (Walmart), Verisk, Dun & Bradstreet, and many more. To learn more about our solutions and how we can help you take control of your data systems, get in touch with us today.
Photo by The Nix Company on Unsplash