Ensuring the integrity of data is important for businesses that rely on it to make informed decisions. Inconsistent or erroneous data can lead to poor decisions, customer dissatisfaction, and diminished trust in data systems. For professionals tasked with safeguarding data integrity, data quality testing becomes a crucial process that serves as the gatekeeper for data, ensuring its accuracy, consistency, and reliability. By vetting data through rigorous tests, professionals can prevent bad data from causing ripple effects throughout their organizations.
Whether you're an IT professional keen to safeguard your data assets or a decision maker aiming to bolster your organization's data-driven strategies, this guide will teach you what data quality testing entails, why it's a non-negotiable in the data lifecycle, and how you can employ strategies to uphold the highest data standards.
Introduction to Data Quality Testing
Data quality testing is an essential aspect of managing data assets effectively. In this section, we'll take a better look at the significance and fundamentals of data quality testing and why it's important.

What Is Data Quality Testing?
Data quality testing is an analytical process designed to ensure that datasets meet rigorous standards of quality. It involves validating data against a set of predefined benchmarks for quality, including but not limited to accuracy, completeness, consistency, and reliability.
Just like the quality control processes in manufacturing, where each part is inspected to confirm it meets specified criteria, data quality testing similarly scrutinizes data for errors, discrepancies, and inconsistencies that could render it unusable or untrustworthy.
Why Is Data Quality Testing Important?
The importance of data quality testing can be distilled into a single sentence: high-quality data leads to high-quality insights. Imagine navigating a ship in treacherous waters without an accurate map; such is the disadvantage of making decisions with subpar data. Data quality testing is the cartography that charts a course for businesses to follow with confidence. Poor data can lead to misguided strategies, flawed analytics, and costly errors. Conversely, high-quality data can enhance customer satisfaction and boost competitive advantage.
Understanding the Key Qualities of Data Quality Testing
To safeguard the trustworthiness of data, one must navigate the multifaceted landscape of data quality testing. Each element represents a pillar that upholds the structure of data integrity.
Here, we'll look into their significance and collective contribution to data excellence.
Accuracy
Accurate data correctly reflects the real-world values it's supposed to represent. Incorrect data leads to incorrect conclusions; hence, accuracy in data quality refers to the precision and correctness of data. Testing for accuracy involves cross-referencing data against verified sources or benchmarks to confirm its validity.
Strategies to improve accuracy include implementing data validation rules, routine audits, and employing error-checking algorithms. Acceldata's suite of data observability tools offers comprehensive solutions to monitor and enhance data accuracy, ensuring that your datasets meet the highest standards of precision.
Consistency
Consistency ensures that data appears uniformly across various datasets and systems, which is crucial for maintaining data integrity. It involves testing for contradictions in data values within a dataset or across systems. For example, consistency checks confirm that a "customer ID" matches across different databases, preventing mismatches that could cause significant data issues. Automated tools from Acceldata streamline this process, making it easier to identify inconsistencies and maintain data harmony, thus enhancing overall data quality management.
Relevance
Data relevance ensures that the information serves the current needs of the business. Irrelevant data can mislead and distract from critical insights. Data quality testing helps to ensure that the data you have is applicable and beneficial for the task at hand by evaluating datasets against the current business objectives and operational context. It's the act of removing outdated or unnecessary data—akin to pruning a tree to yield the best fruit. Tools and platforms that Acceldata provides help organizations prioritize data relevance, enabling them to focus on information that drives actionable insights.
Completeness
Completeness in data quality implies that all necessary data fields are populated and that datasets are devoid of gaps that could lead to incomplete analysis. Data quality testing checks for completeness, which involves identifying missing, null, or unavailable data points that are essential for comprehensive analysis.
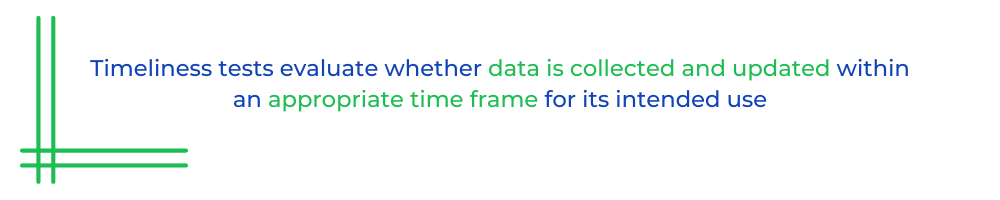
Updates and Timeliness
Data is not static; it's constantly evolving. Timeliness tests evaluate whether data is collected and updated within an appropriate time frame for its intended use. Acceldata's tools automate the monitoring of data flows, providing alerts when updates are necessary. This automation helps businesses maintain the freshness and relevance of their data.
Each of these elements plays a critical role in painting a reliable picture of the data landscape. Through rigorous data quality testing, organizations can make informed decisions based on data that's accurate, consistent, relevant, complete, and timely.
Practical Steps for Implementing Data Quality Testing
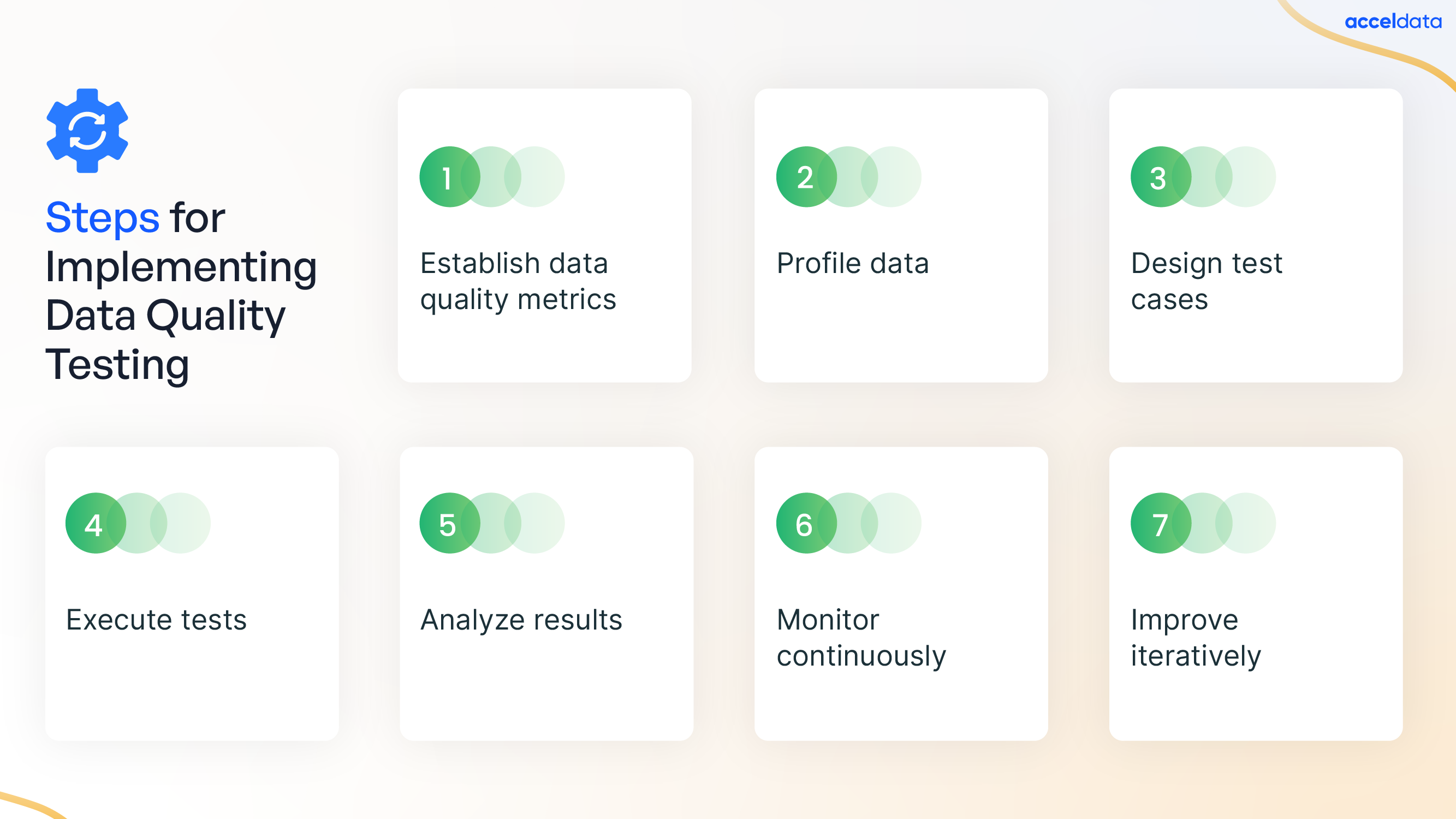
Implementing data quality testing is a planned process that involves several steps. Each step is critical to ensure the reliability of your data. Here’s a structured approach, reflecting the standards you'd find with Acceldata’s solutions:
- Establish data quality metrics: Before you can begin testing, you must define what quality means for your data. Determine the metrics by which you'll measure quality—be it accuracy, completeness, or timeliness. For guidance on which metrics to prioritize, consider insights from resources such as Acceldata’s knowledge base.
- Profile data: This step involves examining the existing data to identify any obvious issues such as inconsistencies, duplicates, or missing values. Tools similar to those offered by Acceldata can automate this process, providing a comprehensive overview of data health.
- Design test cases: Once you identify issues, create specific test cases to check for these problems. Design these tests to be repeatable and automate them for efficiency, similar to the automated monitoring solutions in Acceldata's toolkit.
- Execute tests: Execute the tests on your datasets. You can manually perform this for smaller datasets or use automated systems for larger volumes of data.
- Analyze results: Post-testing, it’s vital to analyze the results and identify the root causes of any issues discovered. This analysis will inform the actions needed to correct and improve the data quality.
- Monitor continuously: Data quality testing isn't a one-off event; it requires ongoing monitoring. Leveraging platforms like Acceldata’s data observability platform can help maintain continuous oversight.
- Improve iteratively: Finally, make iterative improvements based on the insights gained. This might involve refining data collection processes, updating data models, or enhancing data cleaning procedures.
Data Quality Testing Elevated by Acceldata
Embracing our data quality testing approach is intuitive with the Acceldata Data Observability Platform. This platform provides robust tools for top data quality across critical assets and pipelines. Acceldata ensures continuous monitoring and quality checks from source to destination to ensure data quality control. Its advanced analytics and machine learning offer insights into data issues and business impacts. This helps teams prioritize and address urgent data concerns. The platform supports data quality for data-at-rest, in-motion, and for consumption. Features like ML automation, no- and low-code tools, and advanced policies boost team efficiency and precision.
With the Data Observability Cloud, professionals can turn workflows into a data control center. This treats data as the critical asset it is, demanding rigorous management.
Explore how Acceldata can transform your approach to data quality. Learn more about our products and how we can assist you in achieving high standards of data quality for your organization. Check out our knowledge base, participate in our webinars, and read our case studies to see how businesses like yours are leveraging Acceldata to turn data quality into a competitive advantage.