Digital technologies have enabled advanced technologies like cloud, blockchain, artificial intelligence, big data, and machine learning to help the finance industry innovate and revolutionize the way financial services companies compete. As part of this evolution, these companies are creating, storing, and managing increasingly vast volumes of data. In order to operationalize and utilize it, however, they need to ensure data reliability and maximize their data investment.
These responsibilities are addressed with data observability. Though most financial service firms have already started using observability to optimize their data operations, some business leaders lack an understanding of how data observability can mitigate data risks and enhance agility. We’ll outline the specific aspects of data observability and how it provides critical capabilities for organizations in financial services.
Financial Services Data Trends
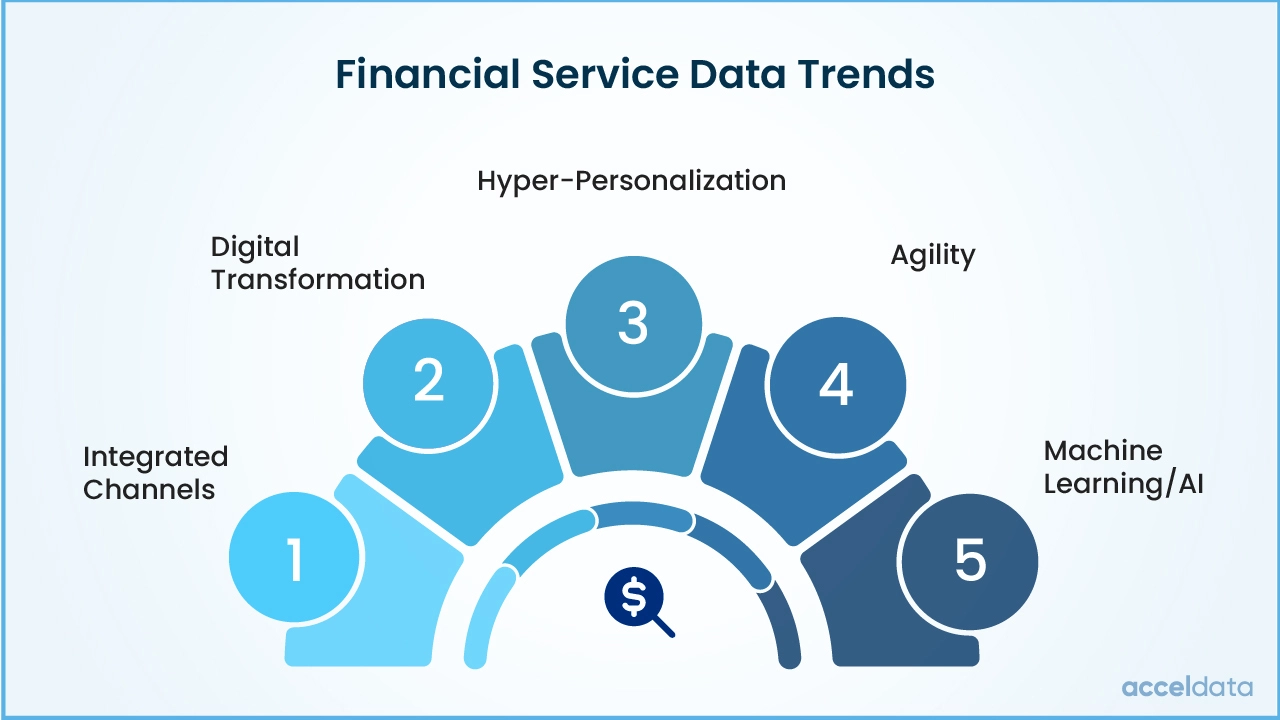
The financial services industry is accelerating its adoption of cutting-edge technologies. With new technologies driving innovation in personal finance and commercial banking, as well as new financial services business types like neobanks and other types of fintech, digitization is reforming the financial sector. The primary trends and challenges of the financial services industry include:
Hyper-Personalization
Gone are the days when one-size fits all services and policies were commonly used in the finance sector. Customers in today’s world are used to having flexibility and more choice in the way their financial products and services are configured. With the accessibility to data on consumer history and behavior, it is now possible to create services tailored to customer needs.
Hyper-personalization allows finance enterprises to leverage and collect information from different sources and use it to deliver targeted services to their customers.
Digital Transformation
Financial services need to reform the way they deliver services, and as the use of mobile banking is increasing, financial service providers need to become more agile. Apart from this, financial service firms are also automating the customer experience with advanced technologies like AI and cloud computing. Digitization allows agility, but it also brings data security challenges, which need to be addressed for a secure experience.
Integrated Channels
Related to personalization, the demand for greater integration among different products and services and across different channels like mobile and online is growing among customers. Integrated channels help to ensure a seamless customer experience, digital competitiveness, better operational flexibility, and efficiency.
Agility
The growing demand for cashless payments and digital services has led to the adoption of more agile ways of banking. New entrants in the finance industry are deploying new business models like open banking, digital assistance banking, and neobanks to offer agility. The existing financial services need to adapt to the constantly evolving technologies to ensure agility, seize new business opportunities, and meet growing customer demands.
Machine Learning/AI
The use of AI and ML in the finance industry is rapidly growing. It is utilized for almost everything from risk analysis to trading decisions. It has changed how banks operate and manage data. Financial service providers are now investing in AI and ML to speed up the process and enhance the overall experience.
“Bad Data” a “Big No” for Financial Services
Big Data is the heart of modern-day trends and technologies in the finance industry. It helps to create sophisticated business models that employ various predictive analysis techniques to cope with the trends.
With proper timely data, businesses can offer innovative financial solutions and products that attract new customers, and new revenue channels, and ultimately lead to increased growth. On the flip side, however, the lack of reliable data can impact decision-making and impact growth. Bad data can be inaccurate, incomplete, inconsistent, and untimely. It can cause unanticipated errors in decision-making. Some primary consequences related to bad data may include:
Inaccurate Analysis
When predictive analysis or data analysis is based on bad data, it can result in misinterpretations and poor decision-making. It produces inaccurate analysis that should never be trusted.
Missed Opportunities
Without reliable data to base business decisions on, enterprises can lose important business opportunities. For instance, poor data can lead to missing out on things like market trends, product improvements, and customer insights. Moreover, inaccurate data can also impact lead generation making it tough to attain determined targets.
Reduced Efficiency
The majority of enterprises require reliable and accurate data to operate optimally. If the data is incorrect or inaccurate, the data teams will have to spend time correcting the errors. Manually it takes a lot of time to deal the data issues and such things drag down overall business efficiency and profitability.
Loss of Income
According to a report by Gartner, many enterprises lose thousands of dollars annually due to productivity loss caused by poor-quality data. It is estimated that bad data costs around $15 million per year to an organization.
Reduced Operational Efficiency
Bad data hinders data teams from innovating with ML and AI models, which reduces their ability to be competitive. Moreover, it keeps digital teams from delivering hyper-personalization impacting the customer experience.
How Data Observability Supports Financial Services with Data Optimization?
Data observability provides the tools and framework required to analyze your data health and infrastructure, optimize your data platform, monitor data problems, and alert and help data teams to rapidly solve issues, Data observability can help financial service firms to enhance all aspects of their data processes including:
Compute & Infrastructure
Data observability allows financial services to maximize the ROI of finance data investments by optimizing their resources, data platform capacity, performance, and costs.
Data Reliability
Observability helps to improve data-based decision-making. Moreover, it also enhances data reliability by improving data quality, performing reconciliation, and identifying data anomalies.
Pipelines
Data observability improves the overall data flow by identifying issues present in data pipelines. Moreover, it uses circuit breakers to stop poor-quality data in tracks and prevents data outages.
Users
Observability delivers real-time insights to all the data personas including data scientists, engineers, administrators, platform engineers, platform leads, and data officers.
Acceldata’s industry-leading data observability platform provides insights and visibility that can help financial service firms to eliminate data incidents, optimize data infrastructure, enable data efficient pipelines, and deliver high-quality data. It optimizes the overall data platform performance.
{{cta-component-vertical}}
Data Observability Use Cases for Financial Services Firms
Financial services require data observability to achieve maximum business benefits from the data applications. Some primary use cases of data observability for financial services include:
Regulatory Compliance
Complete, consistent, and reliable data is crucial for banks and other finance service providers to ensure compliance with different regulations including BCBS, Anti-Money Laundering, and Basil III.
Risk Management
Accurate and reliable data is a must for financial services firms to adequately assess operational, market, credit, and liquidity risk.
Customer Service & Marketing
Coherent and complete customer data helps banks to deliver a seamless customer experience by offering them services tailored to their needs. Additionally, it helps them to cross-sell and up-sell products effectively.
Customer Privacy
Customer data privacy is a critical priority for all financial services firms. To ensure the proper use and privacy of customer data, financial service firms need to constantly monitor the data assets and ensure complete data reconciliation.
Business Execution
Banks are transforming the way they offer services. Therefore they are implementing agile business processes like 24-hours decisions and funding. It requires fresh and timely data for successful execution.
Data Products
Data reliability is an important part of maintaining and offering a comprehensive data product capable of delivering timely, accurate, and reliable data.
Maximize Data Reliability with Acceldata Data Observability
.webp)
Acceldata offers effective data observability insights which make it applicable for various use cases including spend intelligence, data reliability, and operational intelligence, making Acceldata the data observability leader and best choice for enterprises.
Data Reliability
Strengthen your data-driven process by improving the timeliness, quality, and accuracy of your financial data with Acceldata data observability. It offers you multi-layer visibility into your data assets and eliminates data outages to maximize data quality and reliability.
Spend Intelligence
Acceldata offers the most comprehensive data observability solution that can help finance service firms to optimize their data platform spending, allocate resources effectively, and eliminate cost overruns.
Operational Intelligence
Acceldata data observability offers real-time insights of your data platform which can boost your business outcomes. Moreover, it helps to prevent data platform outages and achieve operational excellence to scale your financial data operations on-demand and drive sustainable growth.
Why Acceldata?
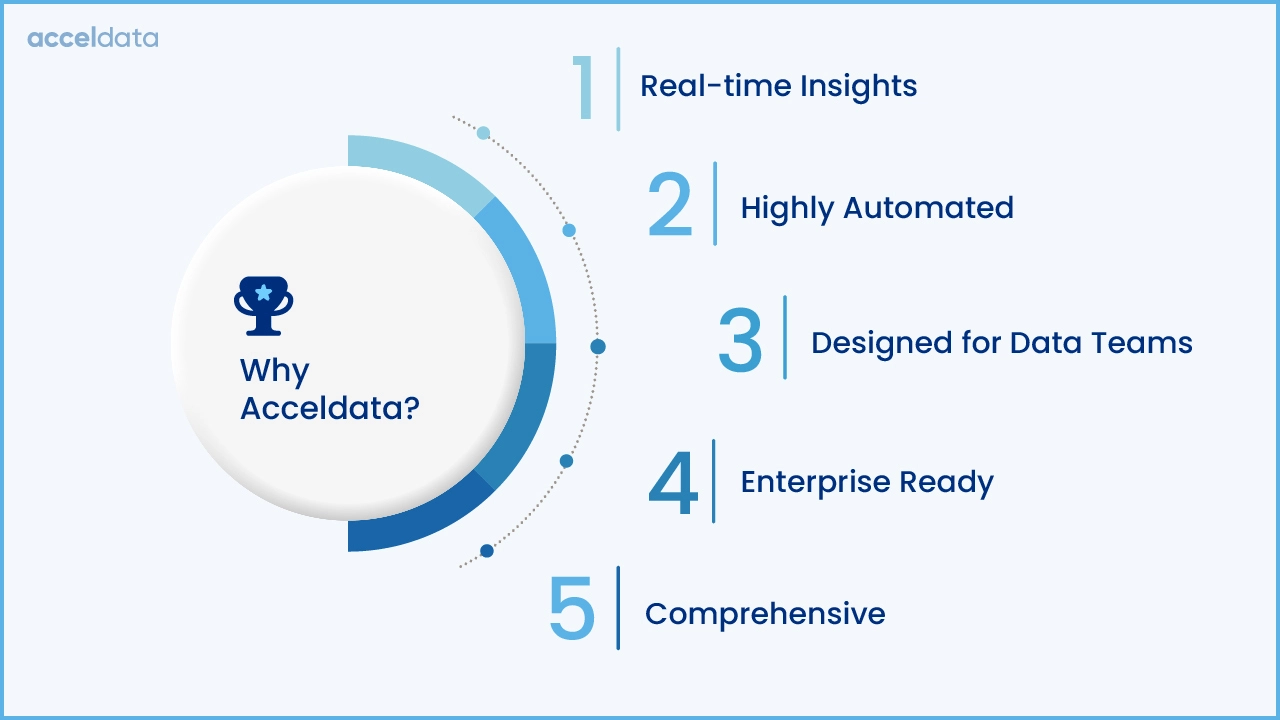
Acceldata offers observability across your data stack to identify, alert, resolve, and even prevent data errors at scale. It covers all four essential areas of data observability: data insights, data, pipelines, data users, and data infrastructure.
Comprehensive
The Acceldata data observability platform is the only one with multi-layer observability across data infrastructure, pipelines, data, and compute. It offers 360° insights, ML-driven optimization suggestions, and quick resolutions to maximize data outcomes.
Enterprise-Ready
Acceldata allows enterprises to boost their data reliability and infrastructure to free up data engineering resources and manage costs. The platform can easily carry out a large volume of data reliability checks on multiple data sets and big volumes of data.
Designed for Data Teams
Acceldata is designed to provide the data teams with insights required to work more efficiently. It eliminates data incident fire-fighting and allows data teams to work on new products that can make finance service firms more data-driven.
Highly Automated
Acceldata’s AI-driven automation allows data teams to easily get the insights they need to manage data pipelines and data infrastructure. It provides automated alerts and composable dashboards to the data teams to keep them updated on data incidents and allow them to resolve issues quickly.
Ensure Financial Sector Resiliency with Acceldata Data Observability
Data is often the primary asset for operational activities. It allows financial institutions to enhance security, reduce costs, and increase productivity. Moreover, it helps them to offer seamless, personalized customer experience across different engagement channels. Data observability allows businesses to ensure data quality, accuracy, and reliability while keeping operational data timely and safe.
If you want to enhance your organization’s observability strategy, we encourage you to book a demo for the Acceldata Data Observability platform.